#26: Diversity in Recommender Systems with Sanne Vrijenhoek
Note: This transcript has been generated automatically using OpenAI's whisper and may contain inaccuracies or errors. We recommend listening to the audio for a better understanding of the content. Please feel free to reach out if you spot any corrections that need to be made. Thank you for your understanding.
What is relevant and what helps people be informed is not the same as just giving them what they would like to read, what they would like to click.
In the news domain there has been a lot of, well, decades of research from social scientists that have thought about the way that news should function in democratic society and how people should be informed.
But then at some point, you know, the technical systems, the recommender systems came around and they kind of completely bypassed all those years of research and just stuck with the traditional conceptualization of diversity, which is ensuring that, you know, the items in your recommendation are not too similar to each other.
I do find that most of the work that appears at RecSys is fairly one-sided. It is about optimizing a metric with some different methods here and there.
You need people that are willing to constantly challenge their own assumptions, to really listen to the other, like what are they hearing? What are they understanding?
And that absolutely goes both ways, but you really do need good intentions in every interaction.
Democratic theory is about, at least the things that I talk about, they are about news.
So you're taking a shortcut if you just think that you can just take that and apply it to your domain.
It really needs to take how to build your own normative framework in a way, which is something that most computer scientists are not used to doing and other disciplines are much more familiar with.
So, let's get started.
Hello and welcome to this new episode of RECSPERTSs, recommender systems experts.
In today's episode, we are discussing the huge and very diverse topic of diversity.
And for this topic, I have invited an expert from academia and we are discussing the multifaceted nature of diversity in RecSys.
That it's actually not that easy to come up with a single definition of it and that it might require a lot of more considerations to come up with a good definition and a good framework.
And I'm very happy for this episode to be joined by Sanne Vrijenhoek from the University of Amsterdam.
Hello, Sonne.
Hi, thank you so much for having me.
Great that you joined and really looking forward to this discussion with you and hearing more about your research, your work at the university and also within the RecSys community.
You have been doing a lot of stuff there and was also very happy to meet you this year in person at RecSys again in Bari in Italy.
So nice to see many people there and I'm very happy that we have the chance to talk about this topic and that you are willing and happy to share about all your research in that field.
So, yeah, as always, I'm going to start with a brief introduction of my guest and then I should definitely hand over to her.
So Sanne Vrijenhoek is a PhD candidate at the University of Amsterdam, where she is at the Faculty of Law, in particular, Information Law, and part of the AI, Media and Democracy Lab.
And she has obtained her master's degree in artificial intelligence.
She has also published many papers at the Recommender Systems Conference, to no surprise, but also at conferences like SIGIR and CHIIR.
And she is also one of the co-organizers of the normalize workshop that took place for the second time as of RecSys 2024.
So, yeah, with that, I would just hand over to you. Please tell our listeners a bit about yourself, about how you got into the RecSys field and what you are doing as part of your research.
Yeah, where to start. So I indeed I do have my master's degree in AI. I think the Netherlands was actually one of the first countries in the world where you had really like dedicated studies in AI.
So I started in 2009, had my master's degree in 2015. But back then, like the whole deep learning chain hadn't even happened yet.
So the type of study that I did was very interdisciplinary. It was really about connecting also sometimes things like psychology, philosophy, really like what makes intelligence.
After I studied, I had done a few different jobs, commercial mostly, but never really found something that stuck until at some point I found what I still describe as the Vegas job description I've ever seen in my entire life.
Which was back then for a one year research project with Natalie Helberger, that's a University of Amsterdam, indeed in the information law department, because they had some ideas about news and news recommender systems and they needed somebody with a technical background to kind of, you know, put those ideas into practice.
And that kind of really appealed to me because I had always been interested in research, but the whole four year PhD commitment always seemed a bit much to me.
But yeah, after one year, we all felt it wasn't fully completed yet. So one year became two years and two years became four years. And then it was like, hey, shouldn't we start turning this into a PhD by now? And well, here I am. Here I am right now.
So yeah, you never actually plan to do a PhD and then you slightly moved into the PhD program because, as you said, you just stuck with it and kind of excited you or your interest grew or what was kind of the point?
Was there even a point or what was it actually that was dragging you so much into that field?
I mean, I didn't have from the get go, like a very strong interest in news. It wasn't like I was driven intrinsically into the topic.
But I think as we continued working, I just saw there was so much depth to the subject and also has like a lot of societal relevance. And also I have to say, like, I have amazing, great colleagues at both the Institute for Information Law at the NDAI Media and Democracy Lab that I really enjoy working with.
So right now I feel very, very dedicated to my work, but it wasn't like a very clear plan from the outset.
For me, it was kind of a bit surprising when I read it for the first time that you are part of a faculty of law, which is something that you wouldn't expect from somebody who publishes papers at RecSys, which is mainly driven by like people.
If you look at the academical side that are part of faculties of computer science. Nevertheless, you're also part of the AI, Media and Democracy Lab, where I guess there are also folks from other faculties.
Are you the only computer scientist at the Faculty of Law or do you sometimes feel like an alien there or how does it feel like? And how is it nowadays? How was it at the beginning?
I was one of the, let's say, the guinea pigs for a more interdisciplinary work, but maybe also nice to explain that information law, it's not like most people, like computer science people think about when they think about law.
Information law means your right to access information. So this is on the one hand, media and news, etc. But it also has to do with issues like copyright, which is now also very current topic with generative AI, etc.
It's also about regulation of things like blockchain. Even within the Institute right now, there are a lot of different people with very different backgrounds.
We have a psychologist, the social scientists that work there, there's somebody that's developing a methodology to use sci fi prototyping to discuss things like regulation.
As a computer scientist, it was a challenge because people come from very, very different backgrounds. And especially if you're just starting out fresh in academia and you actually don't really have a clue what you're doing yet, that it can be a challenge.
So that did take a while in a way to really get started. But we quite quickly started getting other people like external people involved into the project that I would speak to, like, on a somewhat regular basis, people from industry, but also one of my supervisors right now is from CWI, that's the Center for Math and Computer Science in Amsterdam, collaborate a lot with, you know, the people that I do meet at the conferences.
I think the nice part is, is in this way, it's also very flexible, right? I am not stuck on one particular methodology or one particular field in which I have to do my work.
Like my last paper was interview studies. So it gives me a lot of freedom to see like, what does topic we need right now? What does my research we need right now? And what expertise do I need to do that?
Which I think is pretty uncommon in academia, but I really, really enjoy it.
I see. Can I assume that there's also a lot of translation work that you need to perform among the different disciplines?
Yes. And this happens all the time. I am embarrassed to say that it took me a very long time to figure out that IP law is not about IP addresses, it's about intellectual property.
I think I started meetings at the Institute, the law Institute for like almost half a year before the light bulb went off and I was like, oh, this is what I'm talking about.
And vice versa, of course, it also happens. It's very often when I talk about recommender system, I have a particular thing in mind of what a recommender system is.
But I noticed at some point that my colleagues tend to think of social media platforms when they talk about recommender systems.
And you can have very long conversations that way where you actually, or both of you notice that you don't really understand each other, but you cannot really put your finger on what that particular thing is.
So I think they do it in this type of research. It requires a lot from people. It also, you need people that are willing to constantly challenge their own assumptions to really listen to the other.
Like what are they hearing? What are they understanding? And that absolutely goes both ways. But you really do need good intentions in every interaction.
Yeah, yeah. Good intentions in a sense, like not assuming the other person doesn't want to understand you, but that you are maybe just not communicating it properly or that the common ground or maybe the common vocabulary is just missing and that you first need to establish that before like going deeper or talking about concepts that build up on the vocabulary or the basic concepts.
Yeah, exactly. I think in academia, sometimes we have a tendency that we want to show that we're smart and that we know our stuff.
And then sometimes you get into this habit where you're trying to tell people that they're wrong. But when you're doing interdisciplinary research, you kind of need to let that go because people probably aren't wrong.
Like you both are wrong in some way. And you need to find how to get to that common ground.
That's a very good point and something that we should all remember ourselves about. And maybe there are definitely not actually in academia, I guess I would also assume or think from my own experience that this holds true for the industry as well.
Where people depending on also how much trust there is, for example, and how much you actually, yeah, I guess a lot is about trust. And then do I first need to prove myself or can we all assume that we are all competent in our fields and let's say concentrate on the topic and not concentrate on showing off how good we are to each other.
Yeah, yeah. Also, I think that is an environment that needs to be created. And you almost cannot fully do that by yourself. You really need people like Natalie, like my boss that very much from the outset creates a space where there is honest and safe communication where you're not in competition with each other.
And it is one thing also, not only at the law faculty, but also at AI Media Democracy Lab.
In those situations, when you try to explain to that other person what a recommender system is about. I mean, when you first mentioned like people first associate that with social media, I was thinking like, in a sense, they are not very off because these systems are also used in social media, maybe their intent, their objectives are different.
But they kind of borrow from very similar methods, I would say. So in that sense, I would think they're not very dissimilar, but in some certain senses, they are dissimilar. So is it more like discussing recommender systems in the context of news recommendations as their specific domain, what they mean there and what the important aspects are there or what do you do when you try to describe it or where are mental concepts different?
I think it depends a little bit on what you actually are meaning to discuss. If I talk about my research about news recommendation, then we need to have this distinction properly in order. We need to know, are we talking about a recommender system that operates in the context of a local newspaper somewhere and just in there?
Or are we talking about news dissemination happening on Google or on Facebook and Instagram? And then depending on what we need to discuss, then that means that one of the two people in the conversation needs to adjust their core perception.
I mean, I'm not a legal scholar, there's not that much I can say about it. But there's, of course, a lot of regulation happening about social media platforms. So in that context, it also makes sense that these people, when they use the word word, we can use it.
But in that context, it's also fine if you just talk about that particular type of recommender system.
And yeah, this already brings us to the main topic for today's session. And I was actually priming it as diversity in recommender systems, I sometimes already feel like having gone through a couple of your papers, that framing it as this is actually far too narrow.
Because once you dive into diversity in recommender systems, then you basically start asking yourself here, but what does it actually mean? And you have actually done research that is really nice to see, because it goes back to some of the more fundamental questions.
So it felt like, oh, you are really asking the deeper questions. So maybe, can I even introduce you as you are doing research in diversity for recommender systems? Or what would you say about yourself when getting asked, what is your research about? What or which questions are you trying to answer?
Yeah, I think I also adapted answer a little bit, depending on who I'm talking to. So when I talk to a technical audience, I will say, okay, I work on conceptualizing diversity for news recommender systems.
And specifically, my research focuses on news, because in the news domain, there has been a lot of well, decades of research from social scientists that have thought about the way that news should function in democratic society and how people should be informed.
But then at some point, you know, the technical systems, the recommender systems came around, and they kind of completely bypassed all those years of research, and just stuck with the traditional conceptualization of diversity, which is ensuring that you know, the items in your recommendation are not too similar to each other.
So for them, I would say, I work on a more nuanced interpretation of diversity that actually does justice to these normative principles, the normative dimensions of news as well.
If I explained my work to non-technical people, I would explain to them, like, okay, we have technical systems that are taking over the role of human news editors.
But the only thing these systems can do right now is predict what you're likely to click, and that makes them take very different decisions than a human editor would do.
So can we understand how if you had as an individual news reader, if you had a news editor specifically tailored for you, that would see, okay, what does Marcel or Sona have to read right now to have good understanding of the world?
What decisions would that person even make? And it's very different from, can we predict what you're likely to click or what is similar to what you have read in the past?
For me, it sounds like a very, if I would put myself into that position of a person that was not working in the RecSys domain, that would be making perfect sense to me to get it like presented or conveyed in that sense.
Yeah, assume you have a personal news editor that is trying to answer the question how to cater news to your needs, and then you can directly resonate with the idea that just predicting the next article that you are likely to read is not really getting the full picture of what news recommendations in that sense should be about or what someone is actually expecting.
Also, what they could be about, because I think when we talk about news recommendation, we are very likely to end up in a discussion about filter bubbles, right?
We are very scared that these algorithms are locking us in a bubble that we have no control over that we cannot get out of.
But you can also flip this narrative around. If you have an algorithm that locks you in a bubble, you can also have an algorithm that gets you out of it.
And that gives you other things that broaden your perspective. Predicting what you're likely to click is relatively easy, simplifying that a little bit, but really defining how one should broaden their view. That is what my research is about.
And I guess it's also relatively easy to predict because it's a very well researched and very clear objective sometimes. So if we say like predict the most relevant article for an individual, then we are quite easily falling back to all of our standard information retrieval metrics, like precision at K, recall at K, or maybe something like AUC, which we have also seen in one of your most recent works for the RecSys Challenge. But this is definitely something that I would like to come back to later.
I was interested in what you said there, that a lot of the work in news recommendation as well is done based on predicting clicks, because we can measure success there, right?
And it's also important also for news organizations that they get their clicks because they do often need to sell their ads.
But if you start taking things like diversity into account, there's not a very directly a clear return of investment. You also don't know how it's going to impact your sales.
So with that in mind, it is also very easy to just stick to what you know and what the field and what the domain knows. And we'll just keep improving that over and over again.
But it's also not tackling the issues that news recommendations really face. And I talked about this for news, but I'm fairly certain it's also the case with things like music, movie, whatever type of recommendation you want to do.
It's very easy to do the precision at K. It's even harder. It's much, much harder to even get the research started on things that go beyond that.
One additional idea that is crossing my mind as you say this is especially companies that somewhat have to survive in a competitive environment have somewhat to go like the most efficient way, which is sometimes translated into the most pragmatic and easier thing like thinking about the lowest hanging fruits.
And we can get some, let's say, short term success by just going for what's relevant, even though you might hurt yourself in the longer term, but it's something that you can have a very short feedback cycle for seeing like is what I am doing actually having an effect in getting my users to read my content and then also to see ads with which I'm going to earn money.
But you basically neglect a bit what is happening in the long term and in the long term, like you might negatively affect people, society, or even also the retention of people.
Is this something that you would support or what are your ideas?
So I'm not sure if I'm fully answering your last question, but going more on another thought that I had is the fact that the big organizations, they have the money and the resources.
They can experiment with things. But when you talk about media organizations, like individual newspapers, they often do not have the people to carry out extensive experiments.
Smaller newspapers also don't have like hundreds of articles that they publish each day that they can run their tests with. So in a lot of cases, it's also very understandable that just prediction optimization is the norm if there is even anything.
But this is also maybe in a way a place where academia can be of help because we do not have the financial incentives or the need to do that just yet.
We can help with coming up with new things that you could get. Once the idea exists, it would also become easier for the companies to actually put it into production, test it, see what works, etc.
But someone needs to start somewhere.
Yeah, it's a good case for actually the collaboration between academia and industry, as we also see reflected with another of your papers that was in collaboration with with RTL.
And actually, if I go through those authors of the first paper that I would like to discuss again, then I also see that there is at least one person also from RTL, Netherlands, who was part of the authors in that paper.
And maybe this is actually bringing us more talking about, yeah, what are these norms?
But before I would likely about discuss, I mean, we already touched a bit now on the problems or the effects within the news recommendation domain.
But can we even ask about the why it needs diversity before actually specifying diversity?
So maybe let's start with that why and make Simon Sinek happy and start with the why it needs diversity and recommender systems.
I do not know who Simon is, but it is a great question. And I think we should ask ourselves that a lot.
I also want to underline that the why question also gets asked differently depending on the background of the person.
So I have found that indeed with technical audience, I often end up in the why do we need diversity discussion with people from a social science background.
I often end up in a discussion. Why do we need personalization at all?
And it's a different question, but I do think that the answers to both questions are somewhat related.
And we need diversity. We need personalization because right now there is so much information on the Internet or so much news going around that people cannot read everything.
So we also kind of need the recommender systems in order to make sense of everything to bring some sort of structure in there.
I mean, maybe we don't need it, but it could be a very nice way to help people find the stuff that is relevant for them.
But what is relevant and what helps people be informed is not the same as just giving them what they would like to read, what they would like to click.
There are multiple studies in the domain of news recommender systems have shown that when you start having the personalized news recommenders, what happens is that there's going to be an increase in soft news, in entertainment.
Because, yeah, I mean, we all know how clickbait works, right?
You see something that is just outrageous or wild and you are kind of curious about it and you will click it.
Does that mean you were happy after you read it? No, you're not.
But if you're then going to keep reinforcing that by keeping giving people more of that, you're just going to put them off news.
And that is also a trend that we have seen over the past years is that people are engaging with the news less and less.
People are just reading less news because there is too much.
It makes them feel negative.
And maybe the optimistic or naive, however you want to call it, side of me wants to believe that a good news recommender system could play a role there.
And in order to do that, to have a good news recommender system that does reflect editorial values, that does help people be more informed.
You do need something like diversity.
I'm not saying completely let go of personal relevance, but there needs to be more of a balance in there.
So in that sense, these two answers to, let's say, the non-technical people and more like to the technical people are highly connected because many people I could imagine are nodding right now as you talk about personalization, also as support in seeing not only what's relevant, but what is possibly right.
But the question is of what is right is, I guess, even a bigger one.
But let's say to assist in the supply with information in a personalized manner.
But why do we need diversity then?
What does it actually solve?
Which, of course, I guess demands a better definition of the actual problem or the situation as it presents itself to us.
I guess it would be a good reference to hand over to this paper that you have published at CHIIR in 2021, which was called, Recommenders with a Mission Assessing Diversity in News Recommendations, where you actually took a step back and could you maybe share with us what this taking a step back, this is how I would phrase it, has what it has shown and how you then went into diversity from there.
So earlier in this talk, I said, let's imagine that you have a human editor that constructs the news for you.
But that's also a simplification because not every human would make decisions the same way.
And you would make different decisions based on what you want to accomplish with a new selection.
It's a bit of the vision, like how do you envision the role of you as a news organization versus the reader?
Depending on that goal, how you construct your recommendation could be completely different.
This comes from democratic theory.
But I mentioned before with the decades of research into media studies, there were a lot of very smart people, a lot smarter than me that thought about how should news be presented to people?
What should that look like?
What makes for a good new selection?
And Natalie Helberger wrote the core paper that my work then again is based on, which is called democratic role of news recommenders, where she kind of made a description of, well, OK, starting from within democratic theory, there are what we are called like many different models, perspectives that you can take towards news.
And there are more than 40, probably even a lot more of these type of different models.
But in that paper, she focuses on four of them, the liberal model, participatory model, deliberative and critical model.
So between those models, they all have valid goals.
Like, for example, with the deliberative model, what you want to do is to give your readers a very nuanced, balanced overview of the news.
That means that people, all the relevant voices get equal exposure.
So if you were to construct a recommendation based on your deliberative perspective, that is what you would aim for.
People have a neutral overview of everything.
But counter to that, if you take a more critical approach, you would say, well, you are already a person functioning in a democratic society.
You probably already know all the main voices that are out there.
So in our perspective, in this recommender system, we should amplify minority voices so that you can get more acquainted with those.
These are also valid perspectives.
But depending on which one you choose to follow, how you build a recommendation would look completely different.
And that is what we are trying to express with the diversity metrics.
We try to model these more normative goals of the recommender system.
So we do have these four different models that are post out there which have different notions, different, let's say, also priorities.
And you have been laying out these different models in that paper.
But this is one side of the paper because then it's more or the foundational work for talking about the democratic conception of diversity that leans on those different models.
Is that right?
Yeah. So when I started doing this work, and this amazingly great paper that Natalie wrote is completely unworkable for any computer scientists.
It is all it talks about things like democracy and freedom of expression.
And like, yeah, as a computer side, if you read it, you're like, okay, great.
But now what?
Where is the formulas?
Yes.
So that's where I came in.
And what I did there is that I kind of tried to take a critical look at those different models and try to find if there were commonalities and differences in there.
And with that, we came up with a new set or a different set of metrics that will try to express the nuances between those different models.
So that means that for some of the models, you could look at the same metric, but you would have a different expectation of how you thought that metric should behave.
Should this be high or a low value?
So if we go back to the example that I had before about the liberative critical model and how you want opinions to be reflected in those.
What you think you could look at is political voices.
And when you follow the liberative model, you would expect the representation of political voices to be uniform.
Everybody gets equal exposure somewhat.
And for the critical model, you would expect it to be somewhat inverse to how you would expect it based on society.
So let's say as a simplification, say, okay, thinking of political parties.
We kind of want to give less exposure to the parties that are already very prominent because you probably have heard about them already.
And we give more exposure to the to the smaller ones.
I do have to make a remark there that also in this work, we also ourselves come from a certain perspective.
So I am from the Netherlands, which means that I have a certain conception of how politics work with a multi-party system and what gets exposure and whatnot.
One thing about diversity is that it's supposedly very, very different also depending where in the world you are at what point in time you are.
So that also means that any type of diversity metrics like I am not going around and telling people how they should model diversity in their recommendations.
What we need is that they can discuss and make these decisions themselves.
What is valid in my situation, in my use case?
What would diversity look like for me?
And it is still hugely complex, but I believe that we need to start with these simplifications so that we can start having also discussions about things.
If there is something out there, we can have discussions about whether this is good enough or not and where it needs to improve.
But if we do not take that first step with the imperfect metrics, with the imperfect conceptualizations, nothing is really going to happen.
What you do now and also what you do throughout the paper is to take a very modest stance and really not claiming, hey, this is the only truth and this is how everybody should do it.
So one thing that you actually do, you tailor that whole thing to the news domain, which is already a specification that allows you to elaborate more within the context of news.
But it's also the starting point, even though for me this felt much more like just a starting point, but it triggers the discussion and actually enables people a bit more that are seeking for metrics, something that you can take and operationalize to try out.
And I guess sometimes it needs this because even though this normative reasoning is important as the foundation, you also need to have the other side to make, I would say, practical experience to find out, okay, what does it actually translate it into and to find, okay, I get this quantified measurement of something.
What does it mean if I change something? How much is it going to respond to it?
Nevertheless, what you are claiming in those limitations is also, and this is, I guess, what you might be confronted with quite a couple of times when people like read a paper and ask, where are the formulas is something that was great that you took that paper as a chance to remember people about is that formalism trap.
We will say like, yeah, just because I come up with a formula, maybe doesn't necessarily mean that it solves anything. But you actually come up with like five different metrics and people that have worked on diversity before they might know something like intralist diversity that is maybe used quite commonly.
But this is not actually the thing that you are using here. So maybe could you share with us those five different metrics that you come up with as some assisting framework or some some first step there?
So I actually think this is a pretty okay segue into the second paper, the RecSys 22 paper, which was called the radio, they bring to where divergence metrics for measuring normative diversity in news recommendations.
And I do really think like many academics need to come up with more shorter snazier titles.
But at least you do the nice game of coming up with good abbreviations, yeah, like radio. So it's always the radio paper. And it's not only maybe known by that abbreviation, but I also found it was the best paper runner up award at RecSys 2022.
So yeah, congrats to that.
Thank you very much. That was incredibly cool. And also a big surprise to us. Also as of the radio abbreviation, I do have to give credit where it's due. It was Martin Reicher that came up with that one.
So very grateful for that too. But what we did in that paper is that we wanted to find a bit of an, in a way, an alternative to the intralist diversity, like one common base for formalization for the diversity metrics that you could set up in the way that matches your needs and requirements. So if intralist diversity just looks at the items within a recommendation and calculates distance between them, what we do with the radio metrics is that we say that you compare your recommendation or your past reading history to some sort of external distribution.
So coming back to the example we used earlier with political parties, you could say that, okay, we're going to use the distribution of political parties in government as our external distribution.
And then the divergence metrics put express like to what extent is my recommendation similar to this external distribution or not. And I think that covers some of the weaknesses of intralist diversity in its own right.
IOD is also very valid, right? Like we do not want a recommendation that is basically the same item over and over again, even if it has great what we call normative diversity.
But diversity is inherently about you and your situation. So if you're just going to look at the items that are within a recommendation, you are going to miss a very important aspect of diversity, which is what we are trying to cover by having this external distribution.
Also by taking that also into consideration. It is also with IOD, if you talk about diversity in a way that we do, it is very hard to say, okay, well, when is it a good score? Is it 0.7, 0.6?
What does that mean? It's still very dependent on the distance metric that you use. And I'm still not saying that a 0.2 divergence is much easier to express, but at least you know that when the divergence is much easier to express.
When the divergence is 0, the two distributions that you're talking about are completely similar. And when it's 1, they're completely dissimilar.
Yeah, there is a bit of intuitive meaning in there. And also what we kind of a little sidestep here.
One of the things that we also want to do is that we want to use these metrics to start conversations between different groups within a media organization.
We want them also as a vehicle in a way to start discussion between technical teams and editorial teams. And I'm not saying that divergence metrics are from the get-go.
Very easy to understand for people that do not have a technical background, but you can, all these small different decisions that you need to make, like what thing are we going to measure in a recommendation?
What does that need to look like? What are we comparing it to? And is divergence low or high? By kind of breaking the design of your metric up in these little sub-steps, I do hope that it becomes more easily accessible, more easy to understand.
I would like, if we can, also touch at least on these five different metrics that you came up with. Because for me, they actually nicely cover different aspects of diversity.
And then this somewhat relates back to the different democratic models, because as you already mentioned, you would expect different values or put different expectations on where those measurements should move depending on your stance on the democratic models or which you choose.
It resonates with your organization. And therefore, could you walk us or explain to us maybe these different metrics and what they are or should actually capture in terms of diversity?
Yeah, absolutely. Maybe one somewhat disclaimer from the beginning is that what we are also saying with these metrics that we came up with are informed by the democratic models, but they're also examples.
I find it very likely that any organization would say, okay, well, great, Sonja, that you came up with these metrics, but for me, it means something different. That is completely okay.
But we do find that these metrics, they do cover certain nuances of the democratic models, and at least they can be the starting point for discussion.
So if this is not quite what you're meaning, maybe then what is.
But going into the metrics specifically, the first one is calibration, which should be familiar to most of the people working in recommender systems, because it came from the Harald Steg paper calibrated recommendations.
It is also where our idea or inspiration of modeling diversity as a divergence metric came on, where you would kind of express to what extent a recommendation reflects or is tailored to users' personal preferences.
Because also from a democratic theory perspective, it can be completely permissible to just give people what they want to see or expect seeing. For example, it could allow people to specialize in a particular topic.
If I remember correctly, this would then more resonate with the liberal model.
Yeah, I think most recommender systems right now, state of the art, are unknowingly mostly aligned with the liberal model.
Like with the liberal model, you say in the end, the user knows best.
And the trick then is to kind of give them the tools to also express their own autonomy and decision making.
So in that case, if we really want to tailor to the liberal model, what we need to work more on is maybe not explicitly modeling diversity, but it's also giving people control over how they receive and what they receive in their recommendations.
In that sense, we distinguish between calibration of topics, for example, but you could also think about it in terms of style.
Do I receive the news or the articles in a way that it's suitable for me and my specific needs?
So for example, you can give me a pretty complex article about, well, recommender systems, but there is also a lot where I'm not so familiar with, let's say, investment banking.
I know nothing about investment banking.
So if this is the first thing I see about this, like maybe it needs to be a bit more accessible in some way.
And that can also be maybe a bit further down the line.
It's not something that I work on myself, but tailoring to people's needs in that way is also something that from a democratic theory perspective could be considered.
The next metric that we have we call fragmentation, which is more aligned to the discourse of the filter bubble that we also discussed before.
And there is the idea that do people have similar understanding?
Do they see, do they know about the same things happening in the world?
So if you think with the filter bubble that either you get everything in a very one sided way or just some topics that you just never see whatsoever.
With fragmentation, we would try to express what the similarities is between what people see.
And then in an ideal situation, you wouldn't only care.
It doesn't matter if people all read the same articles specifically, but they do need to be aware of similar topics or events.
We actually had a master's student that did a project on this because this is also an NLP problem, right?
Can we identify which news articles belong to the same type of story?
This was actually a, sorry that I step in there because this was something that I was also getting as a new concept when I was reading it in the first paper that you basically talked about that thing like news story chain and to better understand, okay, which news stories belong to kind of the same chain.
And then it becomes an NLP project.
It's more about, and this, I guess you did also in that paper about enriching my existing items or news in that sense with additional data that I need to unlock, let's say the computation or the generation of this metric.
Because if I don't have the data, if I am required to use that data to come up with that concept or to fill it with something, then you basically can't do it.
So is this more like what you mean when you say like it becomes an NLP project there?
Absolutely. I think all of the metrics, everything that we talk about needs a lot of expertise still from different disciplines before it fully can work.
I do not claim in any way that you can go to my GitHub page, just clone the code, run it, and then you have diversity scores.
There are a lot of inherently very different difficult problems that are still to a large degree unsolved.
But the good thing is that there is already a lot of people working on NLP projects.
And I'm very much hoping that if they're interested in part of this, that people will go out and start solving different sub parts of the problem because I cannot do it all by myself.
So if I am more like in this sense, I'm not fully theoretical, like we do show examples of how things could work.
But with the side note that this is a prototype, it's an example, it's not the end solution.
Nevertheless, I guess one should definitely mention here that there are some great GitHub repositories that you also share in those papers where people can go to and take this as a starting point, for example.
I am also still working on refining it. Also this year at RecSys at the INRA workshop, I did a refactored version of our old RecSys paper that I hope is more usable in practice.
It's a living thing. We keep on improving.
This should probably come at the end of the whole podcast, but it's also in a way a call to action to a lot of people that are interested in this to just please get involved because we need help.
And as always, we will add all the references to the resources and also to be able to reach out to you to the show notes.
So if you have ideas, if you want to know more, if you get in contact, then we will definitely facilitate that happening.
Yeah, so we talked about calibration and fragmentation and I'm still sometimes going back to that old one where I like maybe also outline, but you might be doing this anyways.
In that first paper from 2021, you said like there are also existing metrics like for fragmentation, you related to candle, tower, rank, distance or to rank biased overlaps.
But in that radio paper, one thing that was special to it that you derived or used the Jensen Shannon divergence for basically all of those metrics with some adaptions and to make it actually rank aware.
Yeah, this is something that came out of the discussions with my co authors from from that paper Gabriel Benedict and Matteo Canada.
I think they looked at me at some point, it's like, it was like these metrics that you have in your original paper, a lot of them are already divergence based.
So can you not just express all of them as divergence metrics? And then I thought, well, that makes a lot of sense.
So this is how the rest of that paper came about. But indeed, in the first original paper, what was not fully set that all of the metrics always need to be divergence based.
So in the case of fragmentation, I do think that RBO approach makes a lot of sense.
And also in like, if you have a numerical values, which is within the third metric activation or effect, I cannot go back and forth between the names for that metric now and again.
It's perfectly in line with the order that you also post in the paper. So you are well aware of your work.
Yeah, the idea of effect activation is to express more the tone of articles that are recommended.
Maybe you can link this a little bit to part of the discussion where people feel that a lot of the news is very, very negative or very emotional.
But at the same time, I think there have been studies that found that slightly effective news writing does mean that people register it more, they feel it more.
So maybe there can also be a decision there on what do you want it to be? Do all items need to be super neutral or are you allowing for more emotion to be there?
And this is, for example, also part of the critical model that I described before. With critical model, the goal is to challenge existing power structures and imbalances.
And then it can be very beneficial to be able to do that in a more effective tone because you need to resist the powers that be.
So if you're going to enforce that everything needs to be neutral, that's not part of the critical model.
Whereas with the deliberative model, where you want to be very neutral and objective, well, you can discuss whether being truly objective exists, but that is the underlying idea.
Then you would say more, no, we want to focus more on this particular type of content.
And we want to avoid that our recommender system derails and just recommends all the emotional, maybe sometimes clickbaity articles, etc.
Both, again, are valid positions that would lead to different choices in how you construct your recommender system.
All right. So we do have calibration, fragmentation and activation. I guess there are two left, right?
And those are, I think also more related to those topics that we talked about before, the representation and alternative voices, which are both related to viewpoints in a way.
But for me, representation is about what is the viewpoint about an alternative voices is about who has the opinion.
So for representation, there's also a large body of work more related to this in NLP.
It's the viewpoint extraction. There's a lot of very clever ways to think about viewpoints.
But it's also, again, a very hard problem to solve to really find a viewpoint, also in part because, you know, news is a moving target.
And if you're going to train a machine learning model to identify one particular viewpoints in one topic and tomorrow a whole new one pops up, then your old model is not valid anymore.
So this is why in the paper we have chosen to simplify with political parties as a proxy about opinions.
And that is where the discussion that we had before also came from.
For example, you could choose that you want to represent political parties in a uniform way.
You can make them reflective of society or counter that when following the critical model.
And alternative voices is then more about are we giving enough screen time to people from a minority background?
Also, then there's a huge can of worms that you open there then about what is a minority voice.
I think you can hire a completely other sonna to do more research into that.
I am also out of all the things that I am doing in that paper, like the operation that I chose there of alternative voices.
I think now it's a choice. It's probably not the best choice.
But also in this sense, I think it's more about the ideas and what we're trying to express there rather than promising people plug and play code.
And now you can find your minority people and because also here there's a huge discussion that you can have here about the type of analysis that you want to do about the article and also about your users.
Like, do you want to have it explicitly recorded in your database somewhere that this person was of a minority group?
What does that look like? Are you going to say, OK, this person is LGBT and this person has this and this ethnicity?
You may want to do that with the good intentions in some way because you want to promote diversity.
But in this case, I don't think that is a desirable method or outcome.
So also for that particular metric, there's a huge amount of work that still needs to be done before you can have a satisfying way of approaching the presence of minority voices in news.
Which is actually quite an interesting problem for me and already starts to appear as a chicken and egg problem.
So once you bring in that data and actually to increase diversity or have people hurt that were unheard or less hurt before, you kind of make that explicit and then it actually becomes accessible for other purposes that might be undesirable.
But it's actually knowing it is necessary to perform diversity but can actually also harm diversity.
So are there I'm knowing that I'm kind of leaving a bit our pathway here, but I guess it's a very interesting thing that you are bringing up there.
Is there any like research or work that tries to solve this problem with kind of a proxy of this could help or just not actually focusing on protected attributes, but nevertheless doing kind of if we want to say so the right thing?
I'm thinking there's nothing that comes to mind immediately.
And I'm very sorry if I'm bypassing somebody at this point.
And if people do have work on this, please, please reach out because I do think this is incredibly relevant.
I do know that, for example, my colleagues at the AI Mid-Nemor They are doing work on directly interviewing people, people from minority groups about how they experience things.
So maybe in a way, if we want to solve this issue, it's not tech solutionism.
Maybe there isn't a tech solution to this.
Maybe it needs to be done in different ways.
In this case, maybe the best thing that you could do is just hire more diverse journalists and editors to make sure that the content gets produced and not something that your recommender system is going to cover.
And maybe you can have something that could serve as an evaluation metric, but in that case, probably not as optimization if you get distinction there.
Have something internally so that you can check your own output, what is being produced, but not as the end goal.
This is also just something that we are thinking about, or we should be mindful of the fact here that we are in the end, I am, I speak for myself, I am a white person from a rich country that is taking a position on something like diversity and minority voices.
In many ways, I am also from a technical background. I am in many ways not equipped to make statements about those things.
I can give ideas or maybe set up some lines of research, but at some point there is also only so much we can do.
And we also have responsibility that way there to see where our own expertise really ends.
Yeah, nevertheless, I guess it's totally okay and fine to admit what you admitted, but also to say like, we are still, or we also as privileged people do have like the right, at least reason about it.
Like I would say reason with being modest and not claiming that everything is right or true, but at least say, hey, we are thinking about it and here are some possible answers and maybe let's try to adopt them.
Let's further develop them. Let's get in some additional voices and feedback.
I mean, if you wouldn't develop that great research because you would feel like I am not entitled to do that.
It should be people that might be affected to it and then maybe nobody is going to do it or this is going to be delayed.
So I guess in that sense, regardless of who is doing it, I guess it's important that it's being done.
To some extent, yes. One thing I am sometimes afraid of in the context of this work is what you could describe as ethics washing.
That people, that organization would just get their checkbox because they have diversity.
So also part of the work that I am trying to do right now is really think about, okay, what are the base requirements that you need to have in your organization as a researcher, etc.
For something like these diversity metrics to do what it is intended to do, which is make, from my side at least, I mean, almost a bit arrogantly speaking, I hope that it makes the world a better place.
You know, that is the end goal, but I'm trying to get to this list of like, how can you get there and not have it be tackled for, I don't know, nefarious reasons.
Yeah, yeah. I will definitely get back to that ethics washing with regards to another paper possibly.
But maybe first, let's maybe conclude with that paper because having this normative metrics or not this normative metrics, these norms, deriving those more or less qualifying metrics from those and saying like, yeah, but they are not rank aware.
So let's write a paper to make them rank aware, use a, let's say, common divergence metric for it to operationalize this.
And then the next thing that you did is actually not only to derive those, but actually also to apply it to a concrete data set.
Could you touch a bit on that and the comparisons that you performed there?
Yes. So roughly around the time when we started working on the on the radio paper, the mind data set was published from Microsoft News.
I have since then done some work criticizing the mind data set because in many ways it is imperfect, but it was in a way a huge step forward for news recommendations generally because before that there wasn't all that much out there.
And we use this mind data set to showcase how in a theoretical situation and with all these simplifications and proxies that I also described before for the recommenders with the mission paper, how can you find any meaningful difference in there?
So what we did is that we trained the neural recommender systems that came along with the mind data set.
So that's LSTOR, NRMS, etc. And we compared those to some simple baselines like a random recommender, most popular recommender, and then also ran these diversity metrics on them.
Because I think I mentioned this before, it's very hard if you see a score of 0.4, what does that mean? Is this good? Is this bad?
But if you compare it to something like a random baseline, at least you can draw some sort of conclusions about how your recommender system impacts or changes a certain environment.
Because if you're a random recommendation would show in the context of calibration is a recommendation tailored to users personal preferences if you do it randomly and it gives you a 0.4 divergence.
But with a neural recommender, it should give you 0.2. That means that your neural recommender is much more tailored to users preferences than a random one.
And then you have it's less of a decision that you need to make about 0.2 as a value as much as the differences in the score between this baseline and the recommender that you're actually interested in.
And that is also in a way what we tried to show by running the metrics on the mind data set. It was both, hey, these are the effects of the rank awareness that were added to the divergence scores.
You do find more meaningful patterns if you also account for rank awareness. Also rank awareness doesn't make sense for all of the metrics in all of the settings.
So coming back to the political parties and government situation, there is no rank awareness to add there, but that is just your distribution.
But you would account in the recommendation a bit differently, like the article that's shown in first, second, third, fourth position that would influence the distribution that you built eventually.
We also in the paper took a look at, okay, does it make a difference the length of the recommendation that we look at?
Both of those were to show that the metric in some way does work. It's not just some random number that comes back. It does find differences. It does find differences there.
Actually that comparison between the different algorithms and you have been using the most popular and random recommender in that paper and comparing it to four, let's say, new specific recommendation algorithms that, and I have to be honest, I have never heard about.
So I can't barely LSTUR. I guess I have read before somewhere else, but the other ones I was a bit like, what do they actually mean?
So I hope that I'm not being a total noob here, but maybe I should look them up to be better informed.
But at least I saw that it definitely made sense to use some more relevant algorithms for the news recommendation domain and then actually make those compressions to, yeah, as you said, actually see what does it mean for the different metrics.
And I guess this was another step forward into better understanding how well those metrics work. And yeah, I guess it's a very good source of inspiration for people that are working on this or thinking about how they could operationalize it.
You actually went back to that work or also applied it to something that was a bit newer. And as our listeners know, every year along the recommender systems conference, there's also the RecSys challenge, where we do have an organization that publishes an industrial great data set from a certain domain. And for this year's RecSys, so in 2024, this data set was provided by ExtraBlooded. I hope I'm pronouncing that almost at least a bit correctly, which is a Danish news agency.
You have also with some collaborators engaged in that RecSys challenge. And I also have taken a look at this paper. And yeah, it felt for me a bit like this would be a case where you could at least relate a bit to ethics washing.
I'm not hope that this is not too provocative now, but you're already laughing. So I was just thinking when when I've been reading that paper, I was thinking like, yeah, we want to do something about diversity or push forward the issue of diversity in our challenge. But then we kind of fall back to, let's say, the easy to measure thing, which was AOC in that paper.
And you took that chance and actually wrote about it, criticized it, but I would definitely say like in a constructive manner, also deriving recommendations.
Can you share a bit about that work and what was the idea behind it and what your perception was of that data set and of that challenge and whether it was maybe at least a step into the right direction and not a step backward?
Yeah, so this was a collaboration with me and two people from the University of Zurich, Lucian Heitz, Oana Inel. And people are not familiar with their work yet. I would highly recommend they check it because they built the Informfully app, which is a very nice platform to actually test news recommenders recommendations and a very interesting work.
So initially, our idea was that we wanted to not informfully, but their extension of the Kornak framework and see if we could do something within the context of the challenge about, you know, more of not just evaluating because my first two papers were all about evaluation metrics, but can we do something with optimization towards diversity.
This is how we initially got started. And before I go into anything more, I do want to make a side note here that the paper is critical in a lot of ways, but only because I also know the people that work at extra blood and that they actually wanted it to be different.
They did want a challenge that was more focused on diversity. So that's also why in the paper, when we criticize and we when we provide recommendations, it's not about them as individuals or criticizing the organization.
It is also about the way that the RecSys challenge in general is being set up. And the type of work like also the type of decisions that they had to make. For example, they were interested in having multiple evaluation criteria.
But whether that was time constraints or how it was imposed by the organizers, they were made to choose just one. And that is just, I think, a huge loss, which is kind of reflective of RecSys as a field in general.
I've been at RecSys four times. I'm not sure if I'm fully equipped to comment about trends and, you know, a changing scenery. But I do find that most of the work that appears at RecSys is fairly one sided.
It is about optimizing a metric with some different methods here and there. And now we use a graph and now we do sequential and now we have a and a very huge table with many, many, many numbers.
Yes. And we probably did it on movie lens. But, okay, so that was also my personal pet peeve.
I guess you're not the only one.
No, no, no. Also, like RecSys is absolutely not the only conference that has this problem. I have also seen it happen at SIGIR, ECIR.
Maybe it's also just not conferences for me, but I think in the context of RecSys, which is very specifically recommender systems, also about applications, about real things most of the time that work in, you know, in our world.
I do feel that we have more of an obligation to be more mindful, more aware of the things going on around it, more than the more theoretically focused conference as such as SIGIR, for example.
So I think we, while working on the challenge, we did want to also take this as an opportunity to comment on that dynamic about what is it that we're doing here? Why are we doing it?
And also because the data set that extra provided to the challenge participants was pretty high value. It's pretty good. There's a lot of data in there.
They share a lot of, you know, about the articles, which is not easy because you are dealing with copyright issues here.
It's maybe something that you overlook if you're not familiar with the domain, but many people cannot just go around and share article full text.
In that sense, we just felt that it was a very missed opportunity. They did say in their challenge, we value diversity, but then it's not part of the evaluation metrics.
So what we saw it was that all the participants just, you know, optimize that one AUC metric.
You could have the argument like, okay, well, we don't want it's a challenge. We don't want them to gain something like diversity, but you could also argue that they were still gaining a metric, just a different one.
So yeah, again, we felt it was a missed opportunity to get a lot of smart and capable people with a lot of experience to work on a problem like this.
And instead we just went down the good old familiar path of accuracy optimization.
Yeah. And from that point of view, is your, or would your recommendation be just to say, like, provide some trade off between different metrics or is it even the right thing to have a leaderboard with metrics and then like, say, the best contributions are those who hit those metrics.
I mean, you could also like perform a balance of some normalized metrics and then you would come up with some measures that takes both perspective into account.
Would this actually kind of solve the problem or is it a bit more nuanced about how you would solve it or how would you actually improve beyond what has happened with that very good, very rich data set, but actually with that very narrow and very easy might not be the right thing, but well known.
First of all, I mean, I spoke to the people from XRblad, I actually know them quite well and easy, the organizing the challenge, it's not easy.
There is a lot of work going into this.
One of the things that we also say in the paper that could have been a relatively easy fix because they did have in their evaluation platform, like more standard beyond accuracy metrics, such as ILD.
Of course, in an ideal situation, they would have called me and I would have come and I would have built diversity metrics, of course, but this didn't happen and I also understand that.
But they could have said we are sticking to this ILD metric that we do have and we are imposing some constraints on that because what we also saw in the paper was that for the top performing participants, ILD was very, very low.
You could have already made your challenge more interesting if you had put some basic requirement on that one, like we want there to be at least as much interdist distance as was in our baseline algorithm, which they also provided.
And then, I mean, there is also already a lot of work about accuracy beyond accuracy trade offs.
It wasn't even that people would have had to come up with completely new lines of research within the scope of the challenge.
This was a fairly accessible thing that could have happened.
That, I think, is also something that Rex is as an organization, like the umbrella thing that hosts also the challenge.
They could also encourage challenge organizers to think about these sort of things, like how can you make your challenge a bit more interesting than just this, like just support people in organizing and hosting in that way.
Also recognizing that I may be slightly naive and, of course, everything is a lot of work.
I do want to be mindful of that.
Again, that I really do appreciate the work that has been put into this, but suggestions for improvements because we can always improve.
Definitely.
This is also, I mean, where I think that constructive criticism is totally valid.
And I mean, it's not like that you are saying, like, this Rex is challenge a shit and it was totally developed wrongly.
So this is not something that you say.
And I also don't think that people are perceived as such.
So it's more like saying, OK, what was maybe missing?
How could we set it up differently?
And as you said, and I totally agree with that point is there is a huge historical focus on these standard things.
And I guess it's also just easier to do what you know quite well and what you are quite confident and familiar with.
Nevertheless, this community, even though many are annoyed with these old or not really quickly changing patterns, that there are many people within the community questioning things, criticizing things, how we do stuff.
And maybe sometimes it works slower, sometimes faster.
I remember we both were actually sitting in that old RecSys workshop where we also had a had the pleasure to enjoy a nice talk by Robin Burke.
About the post userist recommender systems or recommendations.
And it was actually very nice because it was a quite different workshop in a sense that we just had this as an inspiration.
And then we just took a lot of time to discuss about what is going wrong, which things should be different.
Nevertheless, there was also kind of a notion then, OK, so what we have been doing like these discussions in this or that manner since years now.
But maybe something is changing and maybe this is already a slight signal that things are changed.
But of course, they won't change from one to the other set up within a year.
But at least if we can move it a bit more and push for it.
And I guess this paper is a good demonstration for it because it was part of the track then.
And you said like, hey, things could have been done differently.
And I guess this is important to also appreciate papers and contributions that don't end up with like metrics chasing, but that also point out these are things we are doing not well so far and that we should do differently for these obvious reasons.
And here are recommendations.
I do have to say that it's something I really appreciate about RecSys community in general, because I do often find that people are open to discussion and also interested in what I'm now going to put myself for one second more on the social media.
Second more on the social science side of everything, what people like me have to say.
But you also see a bit of a split sometimes.
So on the Wednesday keynote, there was this person that describes right now, oh no, what we need for progress in AI is that we need statistics, machine learning and economics.
Ah, it was Michael Jordan, right?
The keynote by him.
And at that moment I asked in the online platform, you could ask your questions, like how he was generally interested in that, like how he saw the role of social sciences and humanities within those pillars.
Do they play any role at all?
And unfortunately, the question wasn't addressed by the speaker himself anymore, but somebody else answered.
What were their words?
They are users of AI at best, no role in developing them, period.
And then I thought either you or me is at the wrong conference, because we are like in completely different worlds.
And while I agree with you that much of the RecSys community is also looking outward towards the rest of the world.
And I am also not entirely sure.
Well, maybe I do need to be at a different conference.
I don't think so.
And I don't want to because I really like RecSys.
But I don't hope that this is the case.
But if this is, I also don't directly see how it could or how it could change.
And this was also one of the things with old RecSys workshops, right?
It's what perspectives are currently go missing.
What do we not hear?
It is also what we in a way try to address with the normalize workshop.
We're more about normative thinking about recommender systems in general, where we very actively try to bring in submissions and also reviewers from different disciplines.
So to kind of foster or create a more interdisciplinary space within the conference.
But it's very hard to actually get people that are not technical to come to RecSys because, you know, there's very little in it for them, honestly, especially if you're going to have people comment that you are a user of AI at best.
Also, this is something.
Yeah, if people have ideas about this, please.
And if there are people with a social sciences, social science humanities background listening to this podcast, do it for me.
Submit to RecSys next year.
We will share it a lot.
So, yeah, please come to RecSys.
I guess it needs more of these diverse backgrounds.
And I totally resonate with what you say.
I guess a lot of work still to be done, but this could also bring us to one of the other contributions that you made this year, which was that paper diversity of what on the different conceptualizations of diversity and recommender systems.
It's the only paper that I actually haven't prepared for today's episode, but I guess or I'm totally convinced based on what I have read so far from your works.
It's definitely worth reading, but maybe you can walk us through what you have been looking into with that paper and maybe also how it ties back to your previous work there.
So with diversity of what I mentioned before is that we use the metrics that we describe their conversation.
They're informed, but they're starting point.
And we still saw also a bit of a mismatch between our academic way of thinking about things, democratic theory, and how things could work in practice.
Like what do people really when we go to organizations right now, how do they talk about diversity in an ideal situation?
If they could do whatever they wanted, how would they incorporate diversity in their recommender system?
So we spoke to three public service media organizations in the in the Netherlands, public broadcaster, news organization, and the library who were all to some extent working on recommender systems.
And we did interviews with them.
Part of the interview was where we made them do a little exercise.
We gave them some candidate items from their own database.
Okay, if you were to make a diverse recommendation out of this, how would you do that?
And then we were not interested in the ordering that they made eventually, but as much as what things that they consider when they looked at the different items.
And with that, we kind of created a sort of taxonomy with different aspects, we call them, of diversity that they would consider, many of which you can trace back the democratic theory and the diversity models that we have.
But it kind of gives an overview and yet again, another conversation starters of people that look are looking to build diversity into their recommender system.
What could you be thinking about?
And my hope is also that this also diverts from just news, a little bit like some things that they talked about would be very specifically about news but other domains could also take inspiration from this taxonomy that we created, or at least it would make it easier to identify where the gaps are.
So if you see that this and this is in this there, you may trigger it more easily and you're like, oh, but this is missing and this is very important to me.
So we should actually be focusing on that.
So I think with those three works combined, they all really underline the message of like one, we have the core metric, the divergence based rank aware metric, that you can substantiate in different ways and that you can have different expectations of in terms of their behavior.
And yeah, I'm not sure if this is the point to do that, but also in future work, what we want to do is see, okay, can we start this process with news organizations?
Can we take them through this whole chain of things so that in the end, we can come at a practical design for a technical design for a diversity metric that they could potentially start putting into into production?
All right. One thing that I definitely have to ask you, are you getting bored with dealing with news organizations or with news recommendations?
Do I sound like I'm bored?
No, no, not at all. But I was actually asking like, okay, all the stuff is around the different notions of news recommendations.
And people might also be asking which of these norms, also of the of the concepts of the metrics you could possibly apply to other domains or where they might play a role.
What is your thinking about that?
Yeah, so this is originally also where the idea for the normalized workshop came from, because people would come to me and ask them, like, I wish you talked about democratic theory, how can I apply that to my domain?
And then I would be like, yeah, well, this is not the right starting point for the pitch for a normalized workshop.
Maybe. Let's do that.
Yeah, okay. But democratic theory is about at least the things that I talk about. They are about news.
So you're taking a shortcut if you just think that you can just take that and apply it to your domain.
It really needs to how to build your own normative framework in a way, which is something that most computer scientists are not used to doing and other disciplines are much more familiar with.
And I'm also not claiming that I am the expert on it.
So in a way, also, the normalized workshop is a bit of, you know, a group of people that are trying to also to figure it out and improve this process within our own methodology and within the things that we do.
I really like that aspect because now I basically made the same mistake or I entered into the same trap of just like taking that frame and just putting it into another domain and say, let's just like copy it.
We don't even need to think about it or adapt it or reason about where it comes from.
But what you just said is, I guess, very, very important. It makes me really thinking, like, what is the normative framework or what are the norms for your domain?
I mean, in the European Union or where we are living, democracy is the standard where we come from.
But nevertheless, it might mean something different or it might have a different importance for like several domains where recommenders are operating in.
Let's say, like, for example, in the social media, I guess the framework that we have here and also its operationalization might play also a very high role.
But let's say e-commerce or music streaming, maybe does it do the same there?
But it's also like domains where you do have different stakeholders and different interests.
So, for example, in the music domain, think about the providers and maybe provider fairness or maybe like in the streaming domain when you deal with popularity or in the e-commerce domain.
So, yeah, I really like what you said. You can't just, I mean, you didn't say copy, but this is how I translate it for myself, like just copy it and simply apply it without further thinking to your domain.
But think about what are the norms because the norms is what this work also started with.
Absolutely. And a lot of the normalized organizers, Lee Michiel, Johannes Küsef, from Exablant, X3, that we talked about before, we come from the news domain.
But Alan Starko, for example, he does a lot of work also on food recommendation.
And then you also start having different considerations, ecological validity.
And also I want to underline that also economic incentives are a very valid aspect also of normative reasoning.
It's not just like, oh, everything needs to be ethics and morality.
There's also practical considerations that you have.
But what we want to trigger also with the normalized workshop is that you start discussing the tradeoffs between these things, knowing that you will never have a perfect solution.
Perfect solutions don't exist. Everything you come up with will be wrong according to a group of people.
But we do want to try to train this sort of constructive disagreement.
When we did normalize the first time, we had a full day and we did a whole half day session where we designed the recommender system with taking normative consideration into account.
We didn't have that much time this year.
But then we put out what we call provocative statements and had people discuss those.
In a way, we are trying to build a community of people that are interested in doing this sort of thing and just trying to have improvement over time.
So how was then actually this year's workshop?
So I assume that you have already had representatives of different domains taking part in this year's workshop.
So you mentioned like Alan Stark who was coming or who is coming from the food recommendation domain or that space.
You had a lot of folks from the news recommendation domain, which other domains were kind of represented and what was the conclusion or the understanding from the discussion that you had in the workshop.
Where you say like, oh, this is another interesting perspective that we haven't thought about yet and where it might play a role, but it needs certain adaptions or kind of what was the conclusion for you and the organizers, but also for the participants of that workshop from the different domains.
So yeah, naturally, we do have a lot of submissions on news because those are the people that know us.
There was somebody that had stuff on cultural heritage.
There was something about synthetic data sets.
Also, we had a very legal paper, which was for people that I did not know.
So I was very proud of that in a way.
If I'm completely honest, before we had to workshop, I was a little bit in doubt whether we had to organize again, not because I didn't like it or didn't think it was beneficial, but because there are a lot of workshops at RecSys that are in a way, about similar topics.
So there was, we talked about before, there's alt RecSys, there's fact track, there's Rex so good.
There is now the Rogan one also had elements of, you know, societal impact and there was just, you know, we're in the end, we're all fishing, we're angling from the same people.
And I sometimes would feel like, okay, if we're all spreading ourselves out, we never get any critical mass or any real community building.
So maybe the best thing to do would be for us to kind of step out.
And we also go to other workshops and continue building the community in that way.
But then I thought that this question that we had were really, really great.
Yeah, also the participants, they seem to share that, that sentiment.
So we definitely want to continue the work on normalize.
We are still a little bit debating whether we should continue organizing it as it is now as a RecSys workshop, or we should try different formats, different places.
I think right now the general feeling among the organizers was, yes, this was really, really nice, really good.
We should do it again.
But if we do, we will probably come up with some other new funky formats to be slightly different from how you expect most of the RecSys workshops to be.
And maybe it would then also be worth having Kim Feik there as speaker to maybe provide a nice lightning talk, because I know that he sometimes can talk also about funny things that make people laugh.
And also still pose interesting questions or problems.
Yeah, yeah, definitely.
Yeah, cool.
So does this mean that you're at least planning or intending to have another installment of the workshop for next year's RecSys?
We're actually having a discussion with the organizers somewhere next week.
So if this talk had taken place afterwards, I could have told you, but I'm not going to speak for all of us.
But we will definitely continue to work on normalize.
Yeah, that I can say for sure.
Wow.
I guess that was definitely a great overview of all your research about which role that plays for the community and also maybe where the community should evolve further.
Sona, have we missed maybe something in that regard or something that you feel like, oh, this is very important for me to stress or to mention, and this is important and I want to highlight it here.
We have talked about this before, but maybe just to kind of repeat it, because this is somewhat the end of the conversation, is that everything around diversity, normative thinking, it's an interdisciplinary effort that needs a lot of people.
There are also still a lot of technical challenges to be solved, like efficient optimization for these diversity metrics.
And I have many skills, but that's not yet at least one of them.
So I really hope that more people will pick up, you know, like I said before, subparts of this problem that we can start solving.
So again, like if people are interested in this, if they want to work on things but do not quite fully know where their efforts should go or what they can contribute, well, chances are I can have some ideas about where those skills are still necessary.
So yeah, for those people, please do reach out, because there is still a lot of work left to be done.
That's, I guess, a very good conclusion. And already bringing me to kind of the wrap up of this episode, I was curious, how was RecSys 2024 for you? Anything that is very memorable, anything exciting apart from these answers on Hoover?
So how was RecSys 2024 in Bari for yourself?
I mean, I think I really, really enjoyed it. And RecSys for me is about the community and about the people that are there and that are also, you know, accessible to talk to.
Also not scared of a good discussion here and there. And as many people that have met me have probably been tested, I am sometimes a bit inclined to start discussions.
Overall, just a very, very positive experience. Yeah, we also said this before, I wish there was more societal oriented work in the main track.
But for me, the things around it, so the tutorials, the workshops, also the challenge. I mean, I did really enjoy everything that had to do with RecSys challenge and the opportunity to talk to people surrounding that. That was really, really great for me.
I wonder why you don't mention the Karaoke session as well.
I will make the channel my inner lawyer now. I will neither confirm nor deny that I took active part in the channel.
Okay, that's definitely a good one. I will accept that. I need to accept that.
I think by now my rendition of Living on a Prayer is legendary.
Maybe this could become a new question that I introduce to the episodes to ask people about their favorite Karaoke song. So maybe you have just started a Rexburg tradition here.
At some point, you can have best of Rexburg's. Maybe ask people to close the interview with a bit of singing.
Oh, yeah, that would be great. And then we release the Rexburg's playlist. That would be definitely a good one.
Maybe not asking for the best singer because I know definitely the person who won't be part of that. And that's definitely me.
But asking about maybe other people that could be featured at Rexburg's. Are there or is there somebody or are there some people that you would like also to listen to as part of this podcast?
I think you have already done a good job. You have had many interesting guests on the show with Michael X-Tran on Fairness with Manel Slocum, Casino Bauer.
Maybe you could at some point really try to go out there with Talk to a Legal Scholar.
You could invite Joao to talk about the DSA. It could be an interesting one or maybe a more qualitative researcher.
I'm trying to think of people that are not directly within my lab because that's also a lot of proximity bias. So of course I like their work.
From my personal perspective, interest would be really nice to have that sort of work featured here.
That sounds good. So broaden the scope a bit there. We'll definitely give that a shot.
Sure. As always, also all the listeners are very invited to get back to me with additional listeners.
That is definitely something that Rexels was also very useful for. The guest pipeline, if I may say so, has filled quite a lot.
So I'm really looking forward to many people to talk about different topics in the upcoming episodes, maybe just as a small teaser there.
We will be having somebody talking about multi-stakeholder recommender systems, but also about psychological or psychology-informed or aware Rexes.
You might already think about certain people in that regard, but I will keep that secret.
And then, someone in the future, you will see the release. But first thing, when you hear this, you will hear the release and a lot about diversity and recommender systems by SONA.
And with that, I do not only have to say, but I want to say I'm very happy that you joined me for this interview.
I guess a lot of great work that you are doing, great research and interesting, critical things.
Also pointing out to stuff that needs to be done, that is still developing. So I really like that.
And also what I admire and appreciate about your work, that it's always so structured. So it's really easy and nice to follow.
Yeah, it was like, oh, okay, this tailors to that concept and this evolves from that. So it was definitely very insightful and great.
So thanks for sharing all of that with the community and with me.
Thank you very much for your kind words and for inviting Vladimir. I really enjoyed being part of it.
All right, great. Then I see it's already evening and we are now on the winter days where it starts to be dark quite soon.
Nevertheless, I will at least give it a try and have a round of running. And yeah, I wish you all the best for the rest of your day.
Thank you very much.
Looking forward to talking to you soon.
Yes, thank you very much.
Bye.
Bye. Bye.
Thank you so much for listening to this episode of RECSPERTS, recommender systems experts, the podcast that brings you the experts and recommender systems.
If you enjoy this podcast, please subscribe to it on your favorite podcast player and please share it with anybody you think might benefit from it.
If you have questions, a recommendation for an interesting expert you want to have in my show or any other suggestions, drop me a message on Twitter or send me an email.
Thank you again for listening and sharing and make sure not to miss the next episode because people who listen to this also listen to the next episode. Goodbye.
Thank you.
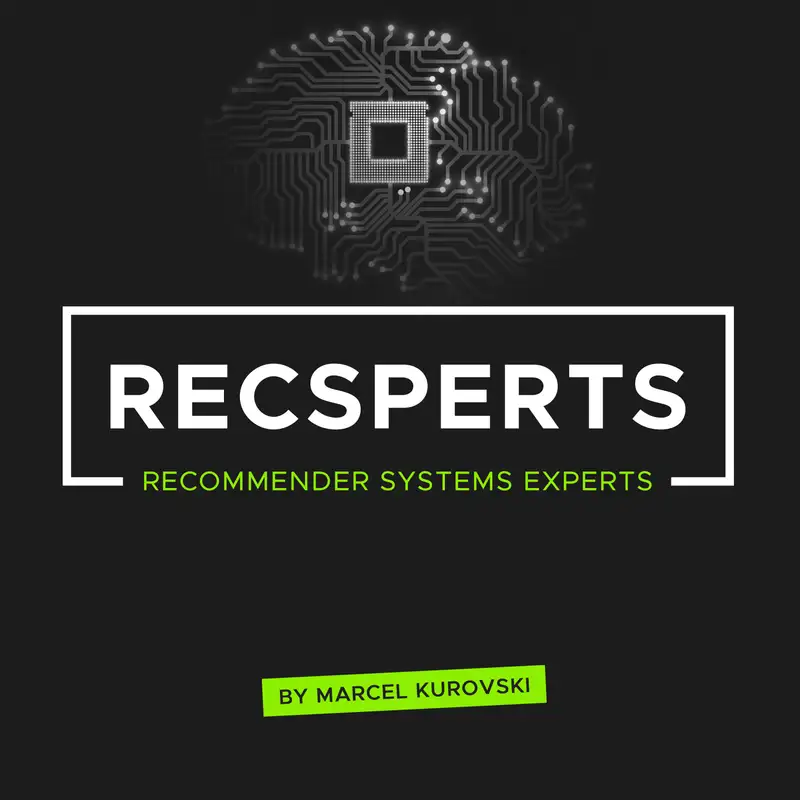