#27: Recommender Systems at the BBC with Alessandro Piscopo and Duncan Walker
Note: This transcript has been generated automatically using OpenAI's whisper and may contain inaccuracies or errors. We recommend listening to the audio for a better understanding of the content. Please feel free to reach out if you spot any corrections that need to be made. Thank you for your understanding.
Personalization is expected by users.
Personalization allows them to get what they want in the small amount of time they have.
Outputs should be impartial, high-quality and distinctive.
You don't want to end up in a position where you have thousands of metrics to the point where you have effectively no metrics because you can't look at them and aggregate them and understand them.
But it is about using a number of touchstones to tell a story about what's going on and to really get a better list to understand.
It's tricky, right? It's like a trade-off between trusting in the statistics but also this kind of softer understanding of what your algorithm is doing at scale.
I don't think we as data scientists, we are not the domain experts, we are not the curation experts.
We shouldn't decide what the good balance between diversity and engagement should be.
It should be those who are the experts in the product, those who are also set the kind of product roadmaps that say, okay, the goals of the organization are those.
There are things that may not be inappropriate today that might start to be inappropriate tomorrow, particularly with current events breaking news and so on.
We could learn how to work alongside non-tech people.
You have just the sort of people that are used to work, you know, to do manual curation and sometimes just you have to find common ground.
You have to find language that both sides can talk, can speak.
Hello and welcome to this new episode of RECSPERTS, recommender systems experts.
For today's episode, I have invited two guests to the show and these two guests are working for the oldest public service media organization.
And some of you might already guess what we are talking about and you are right.
It's the British Broadcasting Corporation, short the BBC.
And for this episode, we are going to talk about how the BBC does personalization, where the BBC provides recommendations.
We will also talk about editorial versus or with algorithmic recommendations and also about the challenges of providing recommendations as a public service media organization, which is a bit different from private media organizations.
And for this episode, I'm very happy to be joined by two experts from the BBC.
My first guest today is Alessandro Piscopo and along with him, I welcome Duncan Walker to the show.
Hello.
Hello. Thank you very much for having us.
Yeah, nice to have you on the show.
And I will soon hand over to you and first start with a short introduction of both of my guests today.
So Alessandro Piscopo joined the BBC as a data scientist in 2019 and is now a lead data scientist in personalization and search.
He originally was coming from a totally different direction with a master in arts and classics and later on moved to data science, obtained his master of science from the University of Amsterdam.
And later on with a PhD in computer science from the University of Southampton working on Vicky Dater.
He is also a co-organizer of the Queer Workshop at RecSys and SIGIR and he has also published papers at the RecSys conference.
My second guest today is Duncan Walker.
He joined the BBC in the same year as Alessandro.
So also in 2019 through a R&D graduation scheme and later on transitioned to working on recommendations research and today works on recommendation systems in production at the BBC.
He has obtained his PhD in theoretical particle physics from Durham University and is today a principal data scientist in the iPlayer recommendations team.
This should just provide the short introduction, but I guess both of you can say a bit more about yourself.
So please share with our listeners what makes you excited about recommendation systems and what made you end up in that domain and working for the BBC.
Maybe Alessandro, if you could go first.
Thank you. Well, that starts with a big question.
I would say just what makes me excited about recommendation systems, especially the BBC.
The first thing, well, just possibly just is not needed in this podcast to say that there's a lot of data available, but media content and especially BBC, we produce kind of more than a thousand new bits of content every day.
And so, like, there might be lots of things, you know, treasure tropes, you know, very interesting things, you know, very engaging, relevant stuff for users, our users in our case.
But for users, I think this is one of the cases where technology can really help to connect people who have limited time with the right stuff and use their time the best they can and finding interesting stuff that they wouldn't have found otherwise. And especially in the case of the BBC, it's also about doing that in a way that is aligned with public service values of which I suppose we'll talk about later.
So I think it's, you know, one case where technology can really help and just give users a better experience of online media content.
What made you changing the direction of your career because you first started with that master of arts and classic and have been working in a different direction.
And then later on, you went totally into this data science direction.
So what was maybe the inflection point there or what made you going into that direction?
Well, it is kind of a serendipitous choice. Well, first of all, I was after my master's and some other study I made, you know, editing and publishing.
I worked for two years in a publishing house as an editor. The main issue with that, I was just working on textbooks and it's I've always felt I was missing something about, you know, textbooks are important.
You know, people, just students learn from those.
But at the same time, how just could I influence, you know, like the future of people, you know, what is it that actually is being built now is happening now.
And, you know, and where can I make a difference?
But these at the time didn't yet, you know, materialize into all of this move to determine data science wasn't really used when I moved to that field.
The actual thing that made me move was actually as always possibly love because my partner was Dutch.
I moved to the Netherlands. And as you can imagine, you know, there wasn't much to do for an editor native Italian speaker in the Netherlands.
And after some times I found kind of a scholarship which allowed me to do a master's in the Netherlands.
And I found that very interesting in information studies, which sounded like techy, but not too much.
I say information, you know, not too far away from what I was doing.
And actually it was indeed. And I had to learn programming and just month, et cetera.
But it was interesting. It was exciting. And so, you know, then it came a PhD that I did actually have after having said, OK, studying, that's enough.
I'm not going to, you know, to continue studying anymore.
And then, you know, I ended up doing a PC and that's how I got here.
OK. And why was it to BBC you chose as your employer?
Oh, well, that's not good.
Another kind of certain deepest moment in those lighting door of moments.
Actually, I mean, this is just as I'm saying that because I am at the BBC now.
I always to one person who used to be a data scientist in our team, Maria Pantelli.
And if you're listening to us, Maria, thank you again, because I think she was already working there.
She was a friend of a friend. I was talking to her. She was the second time we met each other.
I was saying, oh, I really would like to just work in data science and work as data scientists.
But I wouldn't like to work for any company.
I would like just to have purpose. And an organization like the BBC, it would be like a good trade off between being a large organization and at the same time having values.
And she said, oh, I'm actually the scientist at a BBC.
I said, oh, well, that's great.
And after a while she contacted me and said, look, there is a new role that has just been opened in my team.
Why don't you apply? And that's how I got where I am now.
Hmm, cool. And now five years later, you're still with the BBC and I guess have collected many experiences that we might then talk about throughout the episode.
And I already like two things or two words that you brought up there, which are serendipity and purpose.
So I guess two things that somehow reflect about or reflect the moments or your personal career, but also something that we can relate to recommender systems in many ways.
So seeing whether the BBC is successful in providing also its users with serendipitous moments and then actually how you translate the purpose or the values of the BBC into something that recommendations or recommendation systems are going to support.
So, yeah, really excited about that discussion.
Hey, Duncan, I mean, I've already also introduced a bit and provided some background about you, but I guess you are the better person to talk about yourself.
So can you also quickly introduce yourself to our listeners and maybe share with the audience what is your excitement and recommendations and how you ended up in the recommendations team?
Yeah, absolutely. I think it probably made sense for me to start sort of coming to the end of my PhD, which was in effectively large scale simulations of what goes on that hadron colliders.
So you're running huge amounts of four trend code to reduce your error bars by very, very small amounts that's taken 10 years. And at some level, it's a really, really interesting, engaging thing to do.
But it was getting to a point, I think, towards the end where the whole idea of generating these theoretical predictions is that you compare them to data and they match. Fantastic.
You know what you're doing if you don't match, if found another particle or discovered gravity effect or something like that.
But then I on a personal level, I looked at the timelines for new experience, new experiments that might come out and it would be 20, 30 years down the road to build a new tunnel in Switzerland for size of, however large and to generate new experiences and new experiments to compare against.
So at that point, I was sort of ready to move elsewhere, but also having enjoyed the simulation modeling component of what I was doing. And at that point, I found, effectively, the BBC's research and development grad scheme with sort of a vague purpose of knowing that I wanted to do research, sort of modeling work, but not... I tend to find the kind of person who tends to find very interesting things no matter what I do. If I do it for long enough, you'll find that the nuggets are really, really engaging.
And the nice thing about that scheme is essentially you do a certain amount of time in a number of different teams and you sort of pick up those experiences. So it's things like object detection for cameras going on and wildlife shoots was one of the projects I did. But the final one was on recommendations on personalization, sort of looking a bit more to the longer term. It was a project that involved, at some level, work with University College London in a joint project through a data science research partnership that was running at the time.
And yeah, I found that nugget of things that I really enjoy and just kept at it, really.
And then transitioned to working a bit more full time, a bit more permanently as you do as part of these schemes.
But I think there was always at the back of my mind the kind of the itch where recommendations, it's a whole field, it's an interactive field. And you can only do so much by offline evaluation without seeing how what you want to do works in the real world.
It's that difference really between the academic side almost and the industry application of it.
How it survives contact with reality almost. And yeah, at that point, I saw there was an opportunity going in the product side to work on recommendations.
And I took it and that was about 18 months ago, 15 months ago. And here I am.
So it's basically that within that R&D graduation scheme with which you join the BBC, that you basically get in contact with different use cases of data science or different, let's say, domains.
And then you basically found that recommendations and personalization was that field that drew your most attention or how can I understand it?
Yeah, absolutely. I was kind of aware of data science, generally machine learning also.
It was in use in certain areas of physics, but I'd never really sort of come into contact, had that personal experience with it.
And so, yeah, it was that real industrial application of it to say, like, we have this need for it. We need it to do this one thing.
Yeah. And I don't know, like, in some ways, I'm a very simple person. I enjoy something. I want to do more of it.
That's usually my motivation for most things in life.
Just with the difference that nowadays, I guess, Fortran code plays less of a role as it did a couple of years ago.
I have to stay quiet about some of the languages I know sometimes.
That's great. Yeah. Cool.
So first, thanks, both of you, that you joined this interview and that we now today get the chance to share with our listeners a bit more about the BBC and recommendation systems at the BBC.
So and with that, I would say let's dive into it.
And first, maybe I would like to get a better understanding of what is actually the offering of the BBC, because I have seen so far that you folks, you have something like that is called the I player, which for me seemed like, oh, this is some kind of Netflix by the BBC.
And you also, of course, do have on BBC dot com news recommendations, sports recommendations, all of stuff.
You have some all you offering. But can you help me?
And I guess you can to make structure of all of these things.
So what are the offerings of the BBC and where in those offerings does personalization play a role?
And where do you use recommendations?
That's another very big question.
Just one thing I would say next fix is a type of I player rather than.
Just look so as I said, it's a tough question because also just paying at the public service and we can come to what that means later.
I think the BBC is very large, much larger than some people expect, especially outside the UK.
So just while everyone knows just we have news, you know, which can be usually just one associated to the news website or the app.
So that's possibly just the flagship service we have.
There is various sports such as we have textual and just possibly also video content in that of multiple formats already.
But we also have in terms of digital products, because, you know, let's say possibly we could start talking about those.
We have a video in demand platform, which is I player.
We have an audio in demand platform, which is BBC Sounds, which includes podcasts.
It includes music, but not as kind of single kind of songs.
It's in the form of music mixes created by DJs and also live radio.
Just you can access live radio from that.
So besides those that are possibly the most well known, there are lots of other websites.
I definitely miss some, but just there's the loss of education like the BBC Bite size, which possibly Duncan will know more about it because it's grown in this country.
But it's a great resource for students from just early age to, you know, to learn, prepare their exams at school.
There is again the whole children services just we have CBC.
So there's also the whole site for children's programs and services.
What else is in there? This BBC Food.
Well, there's the word service, which is very important.
Word service is another I don't know the terminology here.
It might be another division, but it's just a whole part of the organization where BBC produces news and several languages across the world.
And I think it has a very large audience, which might get to half a billion people just accessing sites every week.
No, 400 million something.
It's very large.
The BBC is many BBC.
Again, we can come to that later.
But there is just BBC produces content and distributes produce and distributes content in many formats, many types, many topics, etc.
And so far, we we have developed recommendations for also the most well known of those products and which are like a player, for instance, which are sport, which are BBC sounds.
We have content to content recommenders in place live in many more service sites.
We used to have some kind of recommend their own on the UK news app, but it's been taken offline for the time being.
So we do recommend this for for be personalized, lots of content and lots of those services.
But there could be many more we could expand to one day.
I mean, yeah, yeah.
OK, so if we take the very widespread offering of the BBC, then what I'm getting from this, the main services or offerings where recommendations play a role are basically the player sounds.
It's news where it's currently not running.
But when you say sports, are we then also talking about sports news or about something different or what do you mean when you say sports?
I think it's BBC Sport is news about sports.
Updates about any sports.
There are live kind of live streams, for instance, when there is a football match or rugby match or any sport cricket.
I don't know enough about cricket to know if you can have a live stream because it's embarrassing or it may be very long cricket match, but.
Oh, OK. Duncan is nodding.
Yeah, I think it's these things are these sites are definitely quite integrated.
So the audio live stream, your your eight hours a day of cricket from a match will be part of the sport offering.
So it's not just news necessarily.
It's a bit more integrated, if that makes sense.
I saw that there was one of the papers that you folks published was actually in, I guess it was 2021 and I have it open here, even though I have to confess I haven't read it.
Building public service recommenders lockbook of a journey.
Can you take us and the listeners maybe on that journey and guide us a bit through where did you start with the recommenders and how have you maybe expanded into different domains and where you nowadays.
I don't know if I mentioned already our team was created in 2018.
Initially, I think the remit team was a bit broader because the aim was to have a team very much focus on machine learning that would develop machine learning in-house, but not as an R&D team, but a team that would be engines to be deployed, various services or products.
I'm using this term interchangeably, but by service of product, I mean something as news or sport or player or sounds.
So something like customer facing.
Yes, yes, yes.
Not necessarily because at the beginning it was thought to be machine learning, just kind of posturing the use and the adoption of machine learning.
I think very quickly, throughout 2018, the team worked on its first kind of use case, which was a recommender.
The first recommender that was deployed and built in-house.
So the first kind of example of algorithmic curation within the BBC.
And it was a recommend.
I wasn't there.
I joined after that, but just to find somewhere where I was recommending clips, so short videos to users based on their previous interactions.
And it was deployed in an app called BBC Plus, which has been discontinued.
Also, I don't think it has a very large number of users, but it was a way to kind of lay the foundations for the team.
From the very beginning, it was a cross-functional team.
So there was this idea that we would have not just data scientists and software or data engineers, but there were also, of course, product managers, but delivery managers, editorial, which has been, I think, super helpful for us.
And having all these people just working together and just working on a concrete use case actually enabled the team to identify all the problems, all the challenges that were connected to building recommenders for public service organizations.
That would kind of basically just comply with and follow the editorial guidelines and possibly support our public service values.
And even in terms of the practical approaches to do that, sometimes just thinking about, oh, let's come up with a simple approach that could work.
So what is the data that we've got?
How good is the data?
Just problems that hadn't been addressed previously to build a recommender system in-house.
How has it continued from there?
So was it then that you just had your first recommender and tried to extend it into different domains or you tried to improve it beyond what it was like at that time or how was the journey from that point continuing?
Before answering the question, I'd like to add something about why that first experience was important.
One of the key things was that we could learn how to work alongside non-tech people.
Just editorial people, they are used to work to do manual curation.
And sometimes you have to find common ground.
You have to find a language that both sides can speak and can use.
And so how do you just phrase things as a data scientist that are clear and can be acted on by someone from editorial?
And how can you just express the fact that, well, you are used to maybe arrange like a base of content, you know, like a few dozen pieces of content maximum at the same time?
Just how do we start thinking in terms of thousands of possible pieces of content?
And then on the data science side, this understanding what are the problems that might arise because we are lucky everything has been kind of checked, just produced, you know, editorial, this is guaranteed that quality of the content is good.
But then you can have issues like, you know, two pieces of content recommended together.
And having those pieces of content together might suggest kind of inappropriate.
I don't want to get into examples because then you may have, you know, something just associated like some some sensitive topics.
You can have an article about a murder and then something that is funny.
It's a matter of also taste and there's an organization.
It's just there's a reputation of risk.
That's what we learn about where just, you know, the journey from that first recommender to the subsequent projects.
I think that recommender was kind of almost abandoned very early on.
We didn't iterate on that also because, as I said, you know, the number of users, you know, all that app were very hundred.
It was very small.
But I think the team, I think it was, you know, when I more or less when I was high, you know, expanded.
We started, you know, being getting into a squad structure.
So we had multiple squads.
We started being a lot more aligned with single products.
So there was a squad that from the very beginning was working alongside BBC Sounds to develop a recommender for them.
Now the squad was aligned to New Sport and the World Service.
And I think initially it was these two squads plus an engineering squad that was working on the infrastructure.
I'm getting also 2019 is a long time ago for me.
But yeah, I think at the beginning, we're like three squads and that's where we started.
And iPlayer, according to the folds, much later, just, you know, they joined our team.
It was 2023, I think it was around February 2023.
So that means that the iPlayer team or the people working on the iPlayer like Duncan were then becoming part of the Sounds or also a dedicated team that was working on the iPlayer later on.
Well, I guess I joined sort of as part of this.
Well, as this iPlayer team was sort of being developed, there have been personalization on iPlayer prior to this.
But it was not necessarily built so much as a data science environment.
And so it had kind of been something that worked technically, but maybe hadn't been iterated on and so on as much as it potentially could have.
So I guess last year, it's been a case of folding that in trying to apply the knowledge that has been obtained in some of the other teams to the iPlayer experience and trying to start iterating on version two.
Okay, before I would like to dive into more of the specifics of the recommender set you have nowadays in place and that support your users across the different domains we already laid out.
I would be a bit more interested in that topic that arises in kind of every paper that you folks have published, which is around public service values and that providing recommendations in the context of a public service media organization like the BBC is a different challenge than if I would do so as, let's say, a private streaming service or something like that.
Because there is an additional dominating public mandate that you folks have to follow or to fulfill.
Can you shed some light on what this means and what this is all about and show, given those values, how this actually translates into your work on recommenders?
Okay, I could start with a bit about what public service means. So, possibly this is something which UK audiences are more familiar with, but the constitutional basis for BBC is the Royal Charter, which is something with a box seal actual.
And I think it's signed by the Queen, even the next one would be by the King.
And it is a constitutional basis. It says how I'd like to see object, mission and public purposes sets out also other things. But I think those are just the main one that interesting to talk about now.
These are these mission and public services are kind of describe what it means for us to be just a public service organization. Can quickly read kind of our mission to just make it a bit clearer.
It is to act in the public interest, serving all audiences through the provision of impartial, high quality and distinctive output and services which inform and you can entertain.
I think one key bits of these is the fact that outputs should be impartial, high quality and distinctive.
And let's remind ourselves that the recommendations just generate or kind of determine our outputs, you know, what's put in front of our audiences. It's not just the content. It's just how it is distributed. How does it watch users see when they open a player or sounds or sport depending on where they are on the side.
So this is already kind of suggesting that recommendations should follow those public through the this mission. But also it's about the public service purposes, the public purposes, which is providing partial news information.
To kind of help people understand and engage with the world around them, support learning for people of ages and kind of show the most creative, highest quality and distinctive output.
And reflect, represent and serve diverse communities of the United Kingdom's and will reflect the United Kingdom culture and values to the world.
And I think here possibly just it's good to stress the fact that we want to represent and reflect the diverse communities within the UK. So diversity is just part of our commitment.
And so just given what I said earlier, that's, you know, like, accommodations determine and are part of our output, those public purposes should apply, should be followed by our recommendations.
Or it should be supported. That is a challenge, because all those things are open to interpretation in sentences. When you get to the implementation of those, how do you do this? Just what does that mean in practice? And how do you implement those? Because again, when it comes to, you know, front page, you know, homepage of BBC News, editorial just could manage, could curate the page manually.
And they could say, Okay, just we have this piece of content, let's balance with these other pieces of content. Don't put, you know, those two things next to each other, because it's inappropriate pairing. Don't do it. Just how do you do it and control that that happens when in theory, everyone, every user could have their personalized homepage.
Right. So how do you scale basically?
Yeah, that's a very good question. I think just there are two sides of this approach of these kind of abiding by our public purposes. When it's kind of active, there is kind of, you know, support those and make sure that everything is reflected. And then when it's passive, which it's basically don't screw up, just ensure that there's no reputational risks. And we had once, you know, we had a very good question.
Like editorial sharing the different things they have to worry about. And there's, of course, accuracy and relevance or recommendations, because, you know, high quality outputs, but there's also impartiality, harm and offense, difficult content, you know, you could have sensitive content about self harm, suicide, graphic content, diversity, then you know, you have protected characteristic, you want to show different points of view. So sometimes we just, first of all, there's the approach of limiting the risks.
And so we have whole set of business rules that reduce the risk of having inappropriate pairings, that prevent anything that would make us liable just for breaking any any loan, you know, for any legally relevant action. And there's the other side, that is basically we have processing place to have editorial to review our recommendations. And right now, just you ask, does it scale well? Well, we do have just a very, somehow manual process in which editorial select very often, and at a very high level, then it happens in different ways in different products. But editorial selects possibly different piece of content that are representative of our catalog. And, you know, we can also create personas. And then we generate recommendations, and we have tools that we have developed in house, just help us visualize recommendations, and help us gather feedback. So editorial can, you know, say whether there are inappropriate pairings, can add some, you know, text within some fields. And then we gather all the feedback. And then we have regular meetings where they explain us. So that there's a lot of manual intervention in that in that, and we as a data scientist, I find it very exciting, actually, we have to translate it and say, okay, this is a very weird thing that we need to avoid how with our instruments, with what we have available with a tool we have available, how can we prevent that not happening? And sometimes, you know, like, we, you know, block list some content, because there's no other way to be completely safe. And other times, we do something that is more nuanced. Duncan, do you want to add anything?
Yeah, I think there may be a couple of things as well to mention here in that a lot of these editorial priorities, they're not necessarily static things, they will change over the course of a year, they'll change over the course of the lifetime of the BBC, in broader terms. So there are things that may not be inappropriate today, that might start to be inappropriate tomorrow, particularly with current events, breaking news, and so on.
Also thinking about things like elections in the UK, there are particular constraints around election times, particularly regarding content that covers politics. So these things are also an evolving, how do I put it an evolve, we work in an evolving system of requirements.
And as a result, we also, you know, obviously, we talk and solicit feedback and have this ongoing dialogue with our editorial counterparts, partly to understand what the rationales are, can we generalize and apply these these learnings more widely?
It's not just saying that this article, that this article is inappropriate, it's what's the wider learning that we can generalize from that.
But we also have a lot of manual overrides and sort of not emergency features, but the ability to turn off certain recommendations quite quickly in the case of a problem has been observed that is sort of editorially inappropriate.
And we have to kind of work in that, in that system.
And that might be at the rail level, or a particular part of the product, it might be wider, it might be the entirety of the BBC thinking about certain things recently.
It's not even a static target, I think is what I'm trying to get.
And as a result, you have to be pragmatic around that as well, so that you can act quickly, debrief and then solve the problem in a more general way, given the luxury of a bit more time.
The first time I hear this, it sounds for me like this is a very manual cumbersome work as well.
Also some work where you could learn a lot, because your editors can support you in better understanding what is appropriate and what is not and help you in translating those values that you just mentioned, Alessandro, into something that you can then encode into some objectives or some rules or something like that.
And then you have that constant, let's say back and forth process in which you would like, for example, to develop a first model, generate recommendations from that model, for example, for different seat users or seat items, depending on what kind of recommender it is.
And then offer them or let them have revisited by your editors and then use the feedback to iterate and go back and forth and back and forth.
What makes me thinking a bit is, I mean, in recommenders, of course, we have learned over the course of the past year set relevance and accuracy are not enough and should not be the only goals.
However, they are more or less easy to evaluate on a large scale, given the feedback of users.
So, for example, in the domain of videos and Duncan, maybe you can can shed some more light on that taking into account how much users are completing a video or something like that.
So, for example, there is the German set to have also a public service media provider and they have offered model cards, shedding some light on their models.
And one of the models that is responsible for recommendations for video recommendation, they are they report that they actually take into account those videos that have a 35% completion rate.
So they are very specific about what they use as input data for their models and to go back to that relationship between you as the, let's say, technical or algorithmic experts and the editors as, let's say, the content experts.
What are the up and downsides of this process in terms of iterating quickly on models, but also in terms of maybe evaluating how effective your recommendations are because I mean, if there is a whole bunch of business rules coming on top of something that is algorithmically created, it can also be quite a challenge to actually evaluate what your recommender is doing when there are so many effects taking place afterwards because then you never really know is what I'm evaluating, actually what I'm finally also showing to the customer and how good am I actually approximating what finally happens in reality.
So maybe a bunch of questions, but can you maybe also with some examples, I have a bit deeper into that collaboration between editors and you and what this means for different things like evaluating the effectiveness of recommenders, how fast and quickly you can iterate and so on.
Yeah, it's a really, it's a really hard thing to do, right, because it depends on how you view it is fundamentally what your model is.
And there is a sense in which your model could just be the machine learning component.
But in actuality, the model interacts with society at large is this model with business business logic in it as well.
But not only that, it extends a bit wider as well, particularly when we're working with curated groups of content that are editorial colleagues who know the content very well, say this content belongs together in this category.
And then we recommend from that how we also understand the human in the loop component of what we do, where editorial colleagues will change their behavior, obviously dependent on what the model is doing and therefore kind of start to build part of the complex system.
That is a recommended system end to end.
And it's something that we are trying to improve in terms of how we evaluate things, both online and offline, in terms of working out what it is that we're evaluating.
We are trying to know whether we are evaluating model or the model and human component together alongside with the business logic.
So essentially, for example, with our A.B. test, editorial colleagues might have learned something about our models and start they use them there at all, essentially, in order to try certain things in some ways.
And so they adapt their behavior in a way that might not necessarily be fair across different variants that they might be using.
I might not correlate with the paper that we took during log data and trying to contract these models and evaluate them offline to begin with.
And it's really tricky. We've had this a few times in terms of building out capabilities such that we can put rails dynamically on pages in different places and the complex interplay with the construction of those rows, with how the models behave, what models you choose, what variants you choose.
And it's something I think we are definitely still learning, but what we found is that a quick iteration process, it doesn't have to be a very formal thing where you write contracts with editorial staff.
That gets you nowhere fast, but it's the case about saying having those quick meetings every so often, right?
Given what you show knows, we've done our offline evaluation. This is kind of how it looks. This is kind of the impression distribution. Does that kind of roughly match? Is that in the ballpark? Does that fit? And what you need are tools to be doing?
And if not, we will go away and have that discussion, not only internally, but with them about how they might be able to construct groups of content, say, that might work better with our models, but also vice versa, how we might work our models better to work with content that they want to promote.
And it's tricky because they're often when we're developing a new tool, a new capability, it's a learning experience, not just for us, but for our editorial counterparts. They're not sure exactly what the optimal output of these capabilities might be because they've never used it before. They've never had it available to them.
And so it's a case of really trying to shorten that feedback loop as much as possible whilst retaining guardrails and things like your accuracy metrics to make sure that you're not going completely off base and accidentally generating a random recommender.
And the same with some of these public service values, like catalog coverage. And we have metrics around that as well. And if something keeps coming up, fold it into your, it's just that standard workflow of if there's a problem that keeps coming up that you could have identified.
Fold it into your evaluation process and just use it as a guardrail for what you're doing.
Alessandro, you also want to share something?
Yeah, just first of all, I wanted to make something clear. We possibly we haven't mentioned it when we talk about the tutorial. It's not the same team or the same person. I mentioned we have someone from editorial editors embedded in our team.
But then, you know, when we developed a developer recommender for a specific product, we liaise with editorial from that product. So there are kind of these three poles. Well, there might be more, but let's take only those in the selection.
That are just data scientists. We are the experts about the algorithm and how you can tweak and pull some levers to make recommendations, maybe more towards diversity, maybe just more for relevancy.
There is editorial from our side. There are kind of experts of content and editorial guidelines and public service purposes. But they are also just in their way, experts about recommender systems because they've been working with us for a while. They've been talking with us for a while. They are embedded in the team. So they thought about the editorial side of recommendations.
And then there are the editorial people, editors from other products we developed our recommenders for that are the experts in that domain, that specific product. So they know all they know the onances, you know, they know what they possibly didn't create the content, but they curated the content.
So they know what kind of content can be problematic to just have together. And I think in that triangle, you know, editorial, our embedded editorial actually allows us to translate needs just back and forth from our side to their side.
But another thing is that possibly just, you know, rephrasing what Dan has just said, I think it's, you know, from our side, what we get from editorial is like, yeah, we can have a metric, we can look at different metrics, we can have a counter-metric.
But my belief is product and editorial, product managers, I mean, you know, product management and editorial, those that should, you know, tell us, they should decide, oh, we are okay with this kind of degree of diversity of courage.
You know, just how we just, we would have trade-offs, we'd have some trade-offs between kind of engagement metrics and just coverage.
Just, I don't think we as data scientists, we are not the domain experts, we are not the content decoration experts. We shouldn't decide what the good balance between diversity and engagement should be.
It should be those who are the experts in the product, those who are also certainly kind of product roadmaps and say, okay, the goals of the organization are those, you know, and just the check-outs of the editorial, you know, what are we comfortable with?
Because, again, there are some just public service values we should kind of abide by.
And, yeah, again, possibly just we don't do yet enough in terms of actively supporting those values.
And we are working, if not our team directly, there is a responsible AI team that works towards that, towards supporting, you know, understanding and measuring public service values in our recommendations.
And in other machine learning engines across the organization.
But I don't think the technical side, you know, in this, you know, triangle on this, you know, it's not a triangle. There are many functions, many people.
It's not the technical side. Data scientists should decide what's good. It's about, you know, having it each other than having people say, this looks bad.
Or, you know, you couldn't imagine just how many inappropriate pairing just could happen, like, at some time, even in terms of titles that cost together, you say, oh, no, no, that's not good.
Okay, when you say this, there are a couple of things popping up in my head that I would like to better understand it also used to.
So first challenge them to maybe better understand. One of them is you say, so, yes, you are the algorithm experts and you can help encode the demands of your stakeholders and something that algorithms can optimize for.
However, being the algorithm experts, we are also confident with statistics.
And for me, it's always hard to understand how people can manually evaluate something that is the output of quite a complex mechanism.
And what I mean by this is especially is a difference in manual evaluation of, let's say, user-based versus item-based recommendations.
That's my personal opinion. Using some kind or notion of content similarity and using algorithm that performs that.
So maybe based on topical similarity that is embedded and say, this is my source item, be it a news article or be it some video or whatever, and now show me something that is similar with regards to content.
I would agree that this is easy to evaluate for humans because you kind of have that human notion of what is content similarity.
And then there is an algorithm basically formalizes this and as an output for one or many seed items, I get those other item recommendations that somehow are exploiting that content similarity.
However, when I look at the other side, so, for example, at users and I use user-based recommendations.
So, for example, with collaborative filtering, then I have a user embedding and I want to use that user embedding to come up with item recommendations.
And there I already think it could be already quite hard because this doesn't have to be similar with regards to the content of what the user has consumed because it embeds patterns of co, let's say, viewing or co-consumption behavior, which is, I would say, much harder to understand at first hand when looking at it manually.
So how do you deal especially with those letter scenarios to or how do your editors deal with that that stuff, even though it might be dissimilar from what the user has already consumed can still be irrelevant for that user.
Yeah, so I think that in the iPlayer domain, it is a challenge and it's one we're grappling with.
I think it's also useful to understand our recommendations in terms of the context in which they occur on the page.
And so we will have a number of different spaces on the page.
Only one of those is around full catalog recommendation where we don't have any kind of, I guess, item similarity constraints on what we're doing.
Even if that similarity is at a level of new and trending content, for example, or most popular content on the BBC over the last week or so.
And as a result, we don't tend to have to look purely at the user based recommendations in absence of any kind of item, meaning a huge amount.
It changes as well, dependent on the product we're talking about.
I think it's also worth saying that a lot of these spaces are also quite heavily curated already.
So they're there for a purpose that editorial want to achieve.
And so there might be a relatively limited compared to the entire catalog with the BBC's content pool from which that they are able to pull from and recommend.
Okay, so basically your your your candidate space from which you finally recommend is already, let's say, constrained by editorial mechanics.
Precisely precisely. And that also gives them a lever with which to use our algorithms to promote what they need and so on as well.
That provides at some level a reasonable amount of safety, because by the existence of the item as a candidate in that group, generally speaking, at least from the player perspective, it's very unlikely that they'll have selected two items from that group that are really very inappropriate when put next door.
I don't say that.
Again, this is very much connected. I just say editorial actually are those that help us understanding the kind of experience we want to provide with any rail that is powered by recommendations.
And so they could, depending on the level which we use for recommendations, episodes or programs, or they could help define, you know, even simpler business rule, but saying, okay, this is the experience we want. We want just use it, which is not just uniquely on the fact that we get metrics that score higher.
But it's also the fact that just we know that this is not a good experience or this is the experience we don't want to be associated with this rail, with this place within the page.
They know, for instance, that each rail goes within a page and so other rail that are possibly only just manually curated will provide that type of content.
And so we say we want to avoid that type of content to appear in multiple places on a page.
And of course, some of those things could be then addressed with automated approaches that we would need to refine.
But others, you know, those are we need that kind of domain knowledge to be able to provide that kind of experience.
I think it's also because you want the algorithmic driven experience within the BBC to have the same kind of style to be informed by our editorial guidelines.
And so you want someone with that domain knowledge.
I mean, I wouldn't let a bunch of data scientists decide for all around the world. I wouldn't trust myself.
This is the reason why we are all trying not to trust ourselves, but rather to trust the data in order to see whether the models that we have come up with are somewhat useful for the consumers.
Of course, that feedback can sometimes be misleading and also lead to the amplification of biases.
So it's the same content as narrowing down and consumed more and more might be a sign of relevance, but definitely not something that you folks want to achieve.
So since you want to go in the opposite direction and rather want to see that users are exposed to, let's say, a diverse set of experiences and in order to be consistent with your mandate.
Alessandro, you mentioned these values like being impartial, being distinctive, being high quality.
I guess high quality for most of the listeners might be something they could directly relate with.
I guess it's not only about accuracy, but I would assume it's more related to accuracy.
However, when saying impartial, distinctive, high quality, what are kind of the algorithm objectives you translate that into or how do you translate it?
And might this look different from the domain we are looking at?
So what forms can these values take when we say we are in news or we are in sounds or we are, for example, in the iPlayer?
I should say that we are very, at the moment, the way, just as I mentioned before, this active supporting our values and it's very naive.
It's done and using guardrail metrics. We do have engagement metrics.
And this is possibly most of the interpretation we currently have about high quality recommendations.
And this is the recommendation that are engaging and relevant for just the user.
Because after all, we haven't said at the beginning that the business license fee funded, which means that just audiences, they pay the license fee.
It's not state funded. It's less fee funded.
People just pay the license fee and they get just all the different services we mentioned earlier.
And so personalization is expected by users. Personalization allows them to get what they want in the small amount of time they have.
Because in terms of time, we compete with lots of different, not just other media providers, but social media and people just have other things to do possibly that don't involve being online.
And so that's being high quality.
Now, in terms of being distinctive, I could associate that to what we were saying or just having this editorial saying, OK, this is where the rail and what we want to convey with that.
In each page within our online platforms, editorial selected content is present as well as algorithmically selected content.
So we have a combination of those types of content in every page.
However, in terms of actual metrics, actual optimization for metrics that represent that's measured to some public service values, plus the area of the stage, there's work being carried out within the responsible team, because this is a topic that's interesting, not just for recommendations, but also for other data science teams within the organization.
We have a data science team, for instance, that look more at content publishing and the society of supporting editors and journalists to produce content.
So I always see this as this kind of continuous line that goes from the creation of content and the publication to us, that are the end stage, it is audience facing and distribution.
And the responsible team is actively working just on developing public service metrics that help us, first of all, understanding whether we are actually doing what we aim to do, that is supporting our public service metrics, but also then at the later stage to optimize our content for those values we want to support.
Duncan, maybe more looking into the iPlayer, can you complement this with some examples, how you translate these values into corresponding metrics for the recommenders that you have in place for the iPlayer?
Yeah, so I think at the base level, I say we're at an early stage on this journey, even defining what these things are in numerically can be quite tricky.
But what we started by doing is a while back, looking at the kind of standard metrics that at least speak to the non-accuracy type understanding of what we're recommending, be it serendipity.
I think there are about 20 different definitions of serendipity that are found in various papers in different places.
I can't remember which one we picked, but we measure that and the same with even just things like catalog coverage.
Actually, quite basic things, when you look at them holistically, you really do start to get a better understanding of what it is they're achieving at scale.
The other very valuable thing that we found is in terms of impressions, distributions, obviously.
But even just eyeballing that can very quickly tell you, you can put warnings around it as well.
But if you just have a massive skew in one direction, we are quite obviously not serving up on this service values, let alone in some of the accuracy stuff as well.
And I think there we often have that quite direct conversation going in real time with editorial curation counterparts who have this sort of quite fundamental understanding of how the item itself, content itself relates to the public service.
And certain content will address certain parts of our public service remit and some will think of a documentary compared to a comedy show.
We have these guiding principles which are inform, educate, entertain, obviously a documentary fits into one part, comedy fits into another.
And so understanding that breakdown and when at scale we look at the aggregated impressions, the way the recommendations are looking on mass doesn't fit with the kind of expectations of our editorial curation colleagues might have for what a public service, you know, if we recommend documentaries to five people.
But comedies do however many millions, obviously we're doing something wrong.
So as I say, it's early days, but we're having those kind of continuous conversations and the goal will be and is to constantly update those.
And every time we find an issue or talk to our editorial colleagues and say and they say actually struggle to give examples off the top of my head.
But we're observing these kind of issues and the diversity of genres that you expect on the top of the homepage, say pulling something out of dinner.
That is absolutely something you can operationalize.
You can put metrics around that and then start to measure.
And you don't want to end up in a position where you have thousands of metrics to the point where you have effectively no metrics because you can't look at them and aggregate them and understand them.
But it is about using a number of touchstones to tell a story about what's going on and to really get a better holistic understanding.
It's tricky, right?
It's like trade off between trusting in the statistics, but also this kind of softer understanding of what your algorithms do in scale.
Okay, gotcha.
So far, I've been looking a lot on that editorial or the algorithmic or combined agotorial topic and how we can translate those values into something that we could also send out.
And measure and hopefully optimize for properly to reach those values with support of algorithms.
However, what are other challenges that you face in providing that personalized experience and delivering recommendations across the different products that you have?
So what are those challenges?
Can you share a couple of them?
So, yeah, I can maybe give an example of one that we often find ourselves grappling with, but indirectly and not necessarily realizing quite so often.
Well, we've got a huge reach, right?
The BBC, particularly for iPlayer in the UK, at least.
From the 90 percent of the adult UK population that we reach, obviously, there are large skews in our data towards certain contents and touchstone things that might have happened during the week.
But that engagement is also driven by things that we don't really have access to personalize information on.
You know, we are we exist in a world where there is both video on demand and live streaming services, but also a huge amount of BBC consumption happens.
Not necessarily off product, but on actual TVs and the same with with radios.
And so what we find is that these linear schedules, let's say the news happens at six o'clock every night and people tune in for the news will really, really skew a lot of the training data that we have in ways that is often first order.
So it's often this is on a prime time television.
By virtue of that, it will get a huge number of people going and trying to find it.
But this entangling our understanding of that content independent of the promotion that it's been given, not just on product outside in UK society, can be quite challenging in terms of over recommending things that are just very, very popular.
But it can also we've also found that those things kind of induce really powerful second order effects that might be a bit less visible until something goes starts behaving in an unexpected way elsewhere on product.
So often we have things like on the journey recommendations off the back of one piece of content that you've watched or recommend you something else.
And what we can find is that if certain content gets really very high traffic, we've observed it over the course of days, we can see an oscillatory behavior in other content on product where they can just drive other impressions of other content and clicks on other content, which then gets promoted and becomes very popular.
So it's quite a complex system that popularity will drive.
Yeah, if that makes sense.
It's something we're struggling to grapple with generally.
Yeah.
So and what is it that you that you do about these problems?
So one thing you mentioned is popularity.
Another one that you mentioned is that release cycle of certain recurring formats, like, for example, the news or maybe there might be a TV show which releases once a week.
And you episode, I guess we haven't fully cracked it yet.
It's something where we are definitely working on.
This is actually sometimes where editorial curations will come in quite valuable.
If we notice off the back of one piece of content is driving the second or effects or even the first order.
We can get an editorial counterparts or view this as not being appropriate.
We can remove that from a set of content available to be recommended.
But the other thing that this does, you can go down the route of popularity, debasing techniques and so on, which we can and have explored.
But it becomes quite tricky when we don't have visibility of a lot of interactions with content, a lot of the drivers that go on.
And so it does lead us also to not just fully depend on collaborative filtering.
You can't just throw it into matrix factorization and hope.
You will also need to lean on metadata similarities quite heavily to try and overcome this.
So I think that's certainly one thing that we found does help in sort of reducing the size of the collaborative filterings effect on the final recommendation.
Either directly or indirectly, compared to metadata can help a lot with the with these popularity biases, even in the first order and then subsequently the second order.
And the more you personalize, the more you can introduce these things accidentally.
And so it's a case of being really, really careful and having adequate monitoring set up so that when things go, when distributions start going haywire, you're able to pick it up and address it as fast as you can before it starts affecting the training data of other upstream models and so on.
That's actually a good point where we could add some within RecSperts advertising.
So for the listeners who also actually want to know more about popularity bias in recommender systems, highly recommend going back to episode 19, where I talked to Himain Abdoula Puri and talked about his work in popularity biases and also in techniques to debias.
So definitely give that a try.
Alessandro, in terms of the challenges, what in addition do you have in mind or come across when I'm thinking about challenges today, but also for the future that you want to address and haven't addressed yet?
I think something that's very exciting, you know, it's challenging, but in the, perhaps in the most possible sense, in the sense that we have hard looks to get to where we want to get and we know about those, but it's going to be fun.
We've talked so far about squads and recommender systems aligned to different products, high player sounds and as you can imagine, this kind of suggests there are mainly BBCs.
And this is partially true because currently very often even they are siloed by product and as well as our team is currently aligned to single product.
But we've gained a lot in terms of building relationships with editorial and product managers from those single products.
But there's an intention within the organization to connect up the BBC, having just all around the BBC experience rather than just simply offering it as a set of different products.
And that means that we will move, we need to move towards thinking of BBC as a whole, thinking about just recommending content across different products and just processing, you're looking at user behavior, not just within each product, but across multiple products.
And there are kind of infrastructural and algorithmic challenges to that. Just we are in the process of building a new platform, you know, that will be a common platform for all our recommender systems to their builds by our team.
I have one more team currently working on cross product recommendations.
So meaning, you know, looking at interactions in one product and providing recommendations for different products, looking at recommendations in BBC sounds and recommending relevant content on a player.
And this is the first time we look at those cross product interactions.
I think it's interesting because in large organizations, you see those things that you have to match, you know, us building the algorithm, but also having the space for that.
There's a new space for you on the BBC homepage where users can find all the content in one place.
All recommendations are in one place.
And that means that at some point, you know, the collaboration with the tutorial that we had, you know, that was kind of limited to, oh, just we don't want to see those inappropriate pairings within one product.
We will have to face this kind of things across different products.
So the problem will scale up to the whole organization, to just all formats and types of content.
And I think, you know, just going down the truth, there is so much we can do in terms of, you know, thinking about the context.
We haven't done contextual recommendations, for instance.
But, you know, just if you want to think in terms of like audio content, video content, news content, you want to, you know, you want to give people the right content at the right time and possibly, you know, just recommending a documentary, a very long documentary, just while people are commuting to work and have just limited time to watch it, might not be the best thing.
Or, you know, we might find out a way to say, OK, just add it to your content, you want to listen in the future.
So there is, I think, one of the things we are going to address next.
As I said, we are just at the beginning, we are just working like part of the team has been working on this cross product recommendations.
But I think this is the start of something that at some point, you know, the whole team will be working with this perspective of not just single products in mind, but the whole of the BBC and more integrated experience.
Yeah, I like that you are bringing that up because it was something that I had in my mind from the very beginning when I was preparing for this interview and checking on the different offerings that you have.
There are so many different domains and then even within a single domain.
So let's, for example, say sounds, you have again subdomains like music, podcasts and so on.
So for me, that looks like you folks have already been working on cross domain recommendations within the domains.
But there is, I guess, even more potential to do that really across the whole BBC.
So to have a user representation that tells me about something about their news taste, but then I might find something corresponding within the player or maybe also a podcast.
Those people are interested in that tells them more about politics because based on their news consumption, I found that they are interested more in politics as they are, let's say, in something different.
And this is something that you are already addressing with its own team.
But but sorry, I didn't want to interrupt.
Just wanted to highlight that team's role.
But yeah, go ahead, Alessandra.
What I'm about to say is just kind of personal view and not just any.
There are no plans within the BBC.
But if we go back to what we were saying at the beginning, it is not just a news for type players.
We have food, we have this bite side for education.
We have children.
You can think about at some point just giving this integrated experience where you know that it's about dinner time.
Just you could recommend some BBC food recipe and some music that is, you know, fits well with that or some podcasts, you know, some drama that is set in Italy or in Turkey.
And you recommend the recipe from those places and you have those all around experiences because we have so much content of so many different times that you can think not just about an experience of a single piece of content, but a multiple experience that goes beyond.
As I said, just we have recipes.
Why not?
You know, thinking, oh, just why don't you just try this recipe?
And at the same time, there's this nice drama and it brings you for an evening in Southern Europe and you can have time.
It's not just about enjoying like a piece of content, but having good, just valuable time where you do something and at the same time you listen and you act or just imagine for teenagers preparing their exams.
Just we have bite size to prepare their exams and then you can say, well, we have this documentary about history.
Why don't you watch that?
Because we know that you are interested in preparing this.
You can add more depth.
You can find it even funny.
We have just even remember if it's a podcast or this horrible histories that is for kids and it's about history and it's fun.
So you can really have those kind of integrated experiences that's only an organization with a breadth that the BBC has can provide.
We didn't know that this is far away in the future.
I was thinking we are not there, but hopefully we will get there.
I guess definitely in some of the things that you provide, you are definitely doing a better job than we are right now in Germany.
What's funny, though, is that the German public service media organization has been founded taking the BBC as an example of the World War II, which I just found.
But when I look nowadays and I feel like you are already a bit more, I'm not sure was a unified is a wrong word.
And there were also good reasons in Germany to have that more of decentralization in terms of the democratization.
But nowadays, when I kind of want to consume some political talk shows, I do always have to choose between two different apps because there is the app that A R D I D has and there is the app that ZDF has.
And on your side, it already seems you got it covered with iPlayer and that's it.
And even iPlayer offers different things from different, let's say, local providers, but it's all at least in one app.
And in Germany, it's kind of at least distributed across two apps, which I don't see any value.
I guess that seems like there is quite a lot of work that is still to be done and also great plans and interesting challenges for the future.
Looking a bit more to the to the RecSys community and your involvement there, Alessandro, I also found that you have been a core organizer for the Queer Workshop that has been taken.
And it's also going to take place for the second time as of last year's RecSys.
And I'm not sure, but maybe you can tell us if it's also going to take place for a third time this year.
So what is that workshop about and are you going to do it for a third time this year?
So I can start with the last question.
And unfortunately, we're not going to do it for the third time because the proposal was not accepted this time, unfortunately.
The other question was that there might be different goals for explanations for recommender systems.
But so far, kind of evaluating just explanations, just along with different goals, there have been kind of bespoke matters, different approaches.
But the existing approaches, like, first of all, there are lots of different approaches and not clear which one can be used in different use cases.
But also, and I think I say this on the kind of industry side, it's been hard so far to find something that could be easily reused in an industry context.
So I personally think that explanation will be a passing or something we should explore at the BBC, just because, first of all, they don't just provide transparency, but it can also increase the effectiveness of recommendations, the stressiveness of your recommender system.
But the amount of work you need to put into developing explanations and the amount of work you need to develop, just you need to develop an approach to evaluate all those aspects of explanations, is doesn't match how much this can be prioritized and how much an organization like the BBC, which is large in terms of broadcaster, but not large in terms of the big tech company.
And what we wanted to do with the workshop was just creating a place to bring together practitioners and academics to kind of discuss those things, discuss around goals, around how those have been evaluated in the past, and about how we can come up with approaches that enable organizations at the BBC, but even just our type of organization to evaluate those goals.
Nice. Okay. Yeah. And anything else from the work that you folks are doing or from the challenges at the BBC that you might want to share with the community or something that excites you also about the future, which we might have missed so far?
Yeah, something that possibly might be worth mentioning is that, and this just by some obvious to some people, but just over the year, we'll come to a stage where we build lots of recommender, and we've been able to say just have recommender's live and most of them just maybe see product. But at the moment, we are kind of, I wouldn't say it's a step back, but kind of bring focusing on, okay, just we build this so far.
How do we get common approaches? Because very often, when you are just focused on delivering or determining products, you start just running away and just you build your thing.
And in any, I think in any environment where you have tight deadlines and you want to deliver on some goals, you then maybe just you need a visualization tool and you have a very skilled developer that say, okay, just we can use this.
This is very easy to make. And we have a visualization tool generated by a squad. Then another squad needs another visualization that has slightly different requirements than the other one.
And you end up with another visualization tool for a mentor. And then so what we're doing at the moment is just trying to kind of four different experts. And so far, we've identified four, which is like just model evaluation approaches, model definition approaches, basic construction, and editorial visualization and feedback tool.
Just we want to say, okay, this is everything we've got, we've developed so far. Let's have a look and let's see how just we can come up with just a single approach, which shouldn't be, we shouldn't constrain anyone.
We should easily adaptable, but not too complicated. It could be complex, but not complicated. You know what I mean?
And having tools that we are a team, just only counting data scientists of 14, 15 data scientists within recommendations. I think just you want to start optimizing, rationalizing our approaches to also just enable everyone to say, you know, the people are working possibly on a product or in a type of recommended person time, but we want to enable also people to move to another topic. And we want also to optimize efforts. So that's, you know, again, we have common tools, we have a common way also to define, you know, a model interface.
I think this will be so important, you know, because at the moment we don't have a common model interface, but that will be enabled us. Okay, just we built this model for iPlayer. Can we try it in sounds? Oh, that would be much faster.
Possibly we will need to adapt it because it's different domain. And again, as I said, we want to connect up the whole of the BBC. So just we will look at this cross product interactions and behavior. But having first, we need the tools to do this. And just we we've recently focused on that. I think it's been very valuable.
Yeah, yeah, maybe if I could piggyback on that as well. There's some, some really interesting things about the fundamental understanding of what people do, how people behave with BBC content. So I think by understanding these things is rather than just being solo product things, if we can start to really tease out the question, not to get fundamental theory of all recommendations and user behavior ever. Lovely. Coming from a physicist, that would be nice, but maybe not.
But it will be really valuable for us to know what learnings and what understandings are consistent between our products. I think that is an incredibly valuable thing to really get. But also what is by disentangling that effect, you also for free effectively get the product specifics.
And being able to clarify that and understand, right, we've we've solved this problem is only for this product. However, we've solved this problem. And we can immediately deploy that out and repeat that learning in multiple places. Not only do we get better recommendations, which is what we're all here for. We develop that better understanding of the generalizability of our learning system.
Yeah, by doing that, you learn faster, you move quicker and repeat that over years and years. Hopefully you get further and further faster and faster. So it's learning, it's learning those kind of fundamental similarities differences, I think will be really, really valuable.
Yeah, definitely. I wish you all the best for that learning journey. Sounds like there's still a lot of things to be done. Okay, towards the end of every interview, I'm asking my guests also what they would like to hear more about, especially thinking about people, but it could also be topics.
Is there anyone that you are having in mind or that you would like me to interview as part of experts or any topics that you might want to know more about that we haven't covered so far? I'm always thinking and you just remembered me of cross domain recommendations.
I'm having already a couple of people in mind who I would like to talk about this. But yeah, if there's any person that you want to hear more about or any topic, what would it be?
I don't know if it's about someone in particular, but something will be interesting to hear. I know that many of your guests that I've heard in the past have this academic and then industry experience, but just as a topic, it will be interesting to hear.
Very often you see models and you see there have never been papers published in the topic. You see those evaluations in academic contexts and then you try to use them in an industry context and they are either useless because they don't get the performance you get or they need huge machines to be trained or real world data.
This kind of relationship in terms of what is developed in academia and having insights from someone who's gone through that process and advise. We could share anecdotes as well, but I remember this was when I moved from my PhD to the role of a scientist. You really see that, oh, what I've done so far has something to do with what I was doing in my PhD, but not quite because you know that this scale and you know there are lots of problems, you know, doing things in practice in a real world setting. Very different.
Yes, I guess related to that is the group Milan who do a lot of work on the offline evaluation in academia, but also how we translate that to better understand how these academic models work in practice and in production. I try and follow what they do. It's been very influential, at least in my thinking. That sounds good. We'll put that onto my bucket list. If it's not there already, I have to check. Great, thanks for sharing that. I guess definitely two quite valuable points that we could address in some of the future episodes of RECSPERTSz. Yeah, and with that, we are at the end of the episode and I thank you very, very much for taking part in this and sharing the experience that you have with the RECSPERTSz community. So thank you very, very much.
Thank you for having us. Thank you.
And then I would say greetings. Go out to the UK and have a wonderful rest of the day. Bye.
Thanks you too. Bye.
Thank you so much for listening to this episode of RECSPERTSz, recommender systems expert, the podcast that brings you the experts in recommender systems.
If you enjoyed this podcast, please subscribe to it on your favorite podcast player and please share it with anybody you think might benefit from it. If you have questions, a recommendation for an interesting expert you want to have in my show or any other suggestions, drop me a message on Twitter or send me an email. Thank you again for listening and sharing and make sure not to miss the next episode because people who listen to this also listen to the next episode. Goodbye.
Bye.
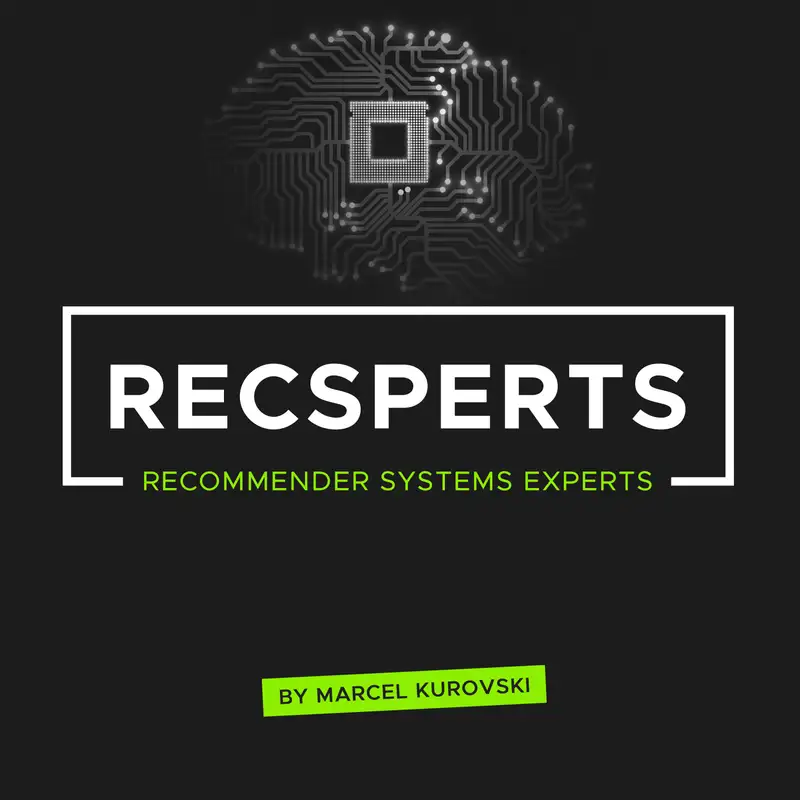