#4: Adversarial Machine Learning for Recommenders with Felice Merra
In episode four my guest is Felice Merra, who is an applied scientist at Amazon.
Felice obtained his PhD from Politecnico di Bari where he was a researcher at the Information Systems Lab (SisInf Lab).
He investigated Security and Adversarial Machine Learning in Recommender Systems by looking at different
ways to perturb interaction or content data, but also model parameters, and elaborated various defense strategies.
In episode four my guest is Felice Merra, who is an applied scientist at Amazon. Felice obtained his PhD from Politecnico di Bari where he was a researcher at the Information Systems Lab (SisInf Lab). There, he worked on Security and Adversarial Machine Learning in Recommender Systems.
We talk about different ways to perturb interaction or content data, but also model parameters, and elaborated various defense strategies.
In addition, we touch on the motivation of individuals or whole platforms to perform attacks and look at some examples that Felice has been working on throughout his research.
The overall goals of research in Adversarial Machine Learning for Recommender Systems is to identify vulnerabilities of models and systems in order to derive proper defense strategies that make systems more robust against potential attacks.
Finally, we also briefly discuss privacy-preserving learning and the challenges of further robustification of multimedia recommender systems.
Felice has published multiple papers at KDD, ECIR, SIGIR, and RecSys. He also won the Best Paper Award at KDD's workshop on Adversarial Learning Methods.
Enjoy this enriching episode of RECSPERTS - Recommender Systems Experts.
Links from this Episode:
We talk about different ways to perturb interaction or content data, but also model parameters, and elaborated various defense strategies.
In addition, we touch on the motivation of individuals or whole platforms to perform attacks and look at some examples that Felice has been working on throughout his research.
The overall goals of research in Adversarial Machine Learning for Recommender Systems is to identify vulnerabilities of models and systems in order to derive proper defense strategies that make systems more robust against potential attacks.
Finally, we also briefly discuss privacy-preserving learning and the challenges of further robustification of multimedia recommender systems.
Felice has published multiple papers at KDD, ECIR, SIGIR, and RecSys. He also won the Best Paper Award at KDD's workshop on Adversarial Learning Methods.
Enjoy this enriching episode of RECSPERTS - Recommender Systems Experts.
Links from this Episode:
- Felice's Website
- Felice Merra on LinkedIn and Twitter
- Adversarial Machine Learning in Recommender Systems (PhD Thesis Final Presentation)
- Workshop on Adversarial Personalized Ranking Optimization at ACM KDD 2021 (awarded Best Paper)
- Adversarial Recommender Systems: Attack, Defense, and Advances (chapter in 3rd edition of Recommender Systems Handbook)
- Information Systems Lab (SisInf Lab)
Thesis and Papers:
- Merra et al. (2020): How Dataset Characteristics Affect the Robustness of Collaborative Recommendation Models
- Merra et al. (2021): A survey on Adversarial Recommender Systems: from Attack/Defense strategies to Generative Adversarial Networks
- find all the papers on Felice's website
General Links:
- Follow me on Twitter: https://twitter.com/LivesInAnalogia
- Send me your comments, questions and suggestions to marcel@recsperts.com
- Podcast Website: https://www.recsperts.com/
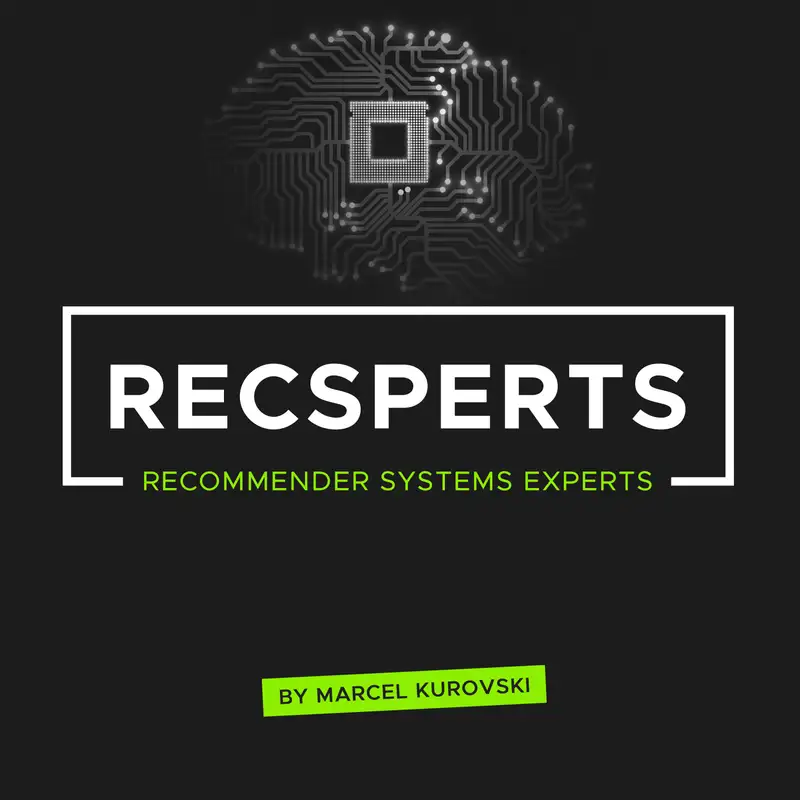